Integrating Artificial Intelligence (AI) within the manufacturing industry marks a significant shift towards more efficient, innovative, and intelligent production processes.
As manufacturing companies strive to enhance operational efficiency and maintain competitive edges, adopting AI technologies is a pivotal strategy.
This transition optimizes manufacturing operations and redefines the traditional quality control, predictive maintenance, and supply chain management paradigms.
AI in use cases in manufacturing: Key insights for business leaders
- AI Transforms Manufacturing: Elevating efficiency, innovation, and quality control across the board.
- Predictive Maintenance & Quality Control: Leveraging AI to enhance precision and reduce operational costs.
- Supply Chain Optimization: Utilizing AI for advanced forecasting and inventory management, meeting market demands efficiently.
- Product Development & Automation: Accelerating innovation with generative design and robotics, pushing the boundaries of manufacturing capabilities.
- Overcoming Implementation Challenges: Navigating through strategic integration and fostering a culture of continuous learning for successful AI adoption.
AI, machine learning, and computer vision are transforming manufacturing, significantly enhancing quality assurance, streamlining inventory management, and setting new benchmarks for excellence.
Relevant statistics
- 93% of industrial manufacturing leaders utilize AI to some extent, leading the charge in adoption.
- European manufacturers, at 51%, with Japan (30%) and the US (28%) closely behind, are integrating AI solutions into their operations.
- Key AI applications include maintenance (29%) and quality control (27%), with quality control being paramount for 59% of respondents.
- Other notable applications: inventory management (44%), monitoring/diagnostics (32%), and customer care (29%).
- Active AI production initiatives stand at 21% within the industry.
Looking forward
As AI revolutionizes manufacturing, businesses are urged to adapt, leveraging AI for operational excellence and innovation. The journey requires navigating through implementation challenges with strategic foresight and a commitment to continuous improvement.
References
AI Usage in Manufacturing - Statista
AI Trends in Manufacturing - Google Cloud Blog
The transformative impact of AI on manufacturing processes
AI in manufacturing transcends automation, offering a wide range of applications that enhance plant capabilities, reduce downtime, and cut maintenance costs through machine learning.
These systems improve quality control with exceptional precision using computer vision and optimize the supply chain by accurately forecasting demand and managing inventory, making processes more cost-effective and market-responsive.
The role of AI in predictive maintenance and quality control
Predictive maintenance is a prime example of AI's utility in manufacturing.
By analyzing sensor data and historical performance records, AI and machine learning algorithms can predict equipment failures before they occur, allowing manufacturing companies to perform maintenance only when necessary.
This reduces maintenance costs and enhances the production process's efficiency by minimizing unplanned downtime.
In quality control, AI systems, particularly those that employ computer vision algorithms, have transformed quality assurance practices.
These AI-powered systems conduct visual inspections on the factory floor with unparalleled accuracy, ensuring that manufacturing standards are upheld without requiring extensive human intervention.
AI's contribution to supply chain management and operational efficiency
AI technology is crucial in refining supply chain management, offering solutions significantly impacting inventory control, warehouse management, and overall supply chain optimization.
By leveraging AI algorithms to analyze market trends and procurement data, manufacturing businesses can achieve precise demand forecasting, optimizing their supply chain to meet market demands efficiently.
Additionally, AI systems enhance operational efficiency across the production line, from the assembly line to the final stages of the manufacturing process.
This is achieved through advanced manufacturing technologies, including robotic process automation (RPA), deep learning neural networks, and natural language processing, which collectively improve manufacturing facilities' production capacity and efficiency.
Core AI use cases in manufacturing
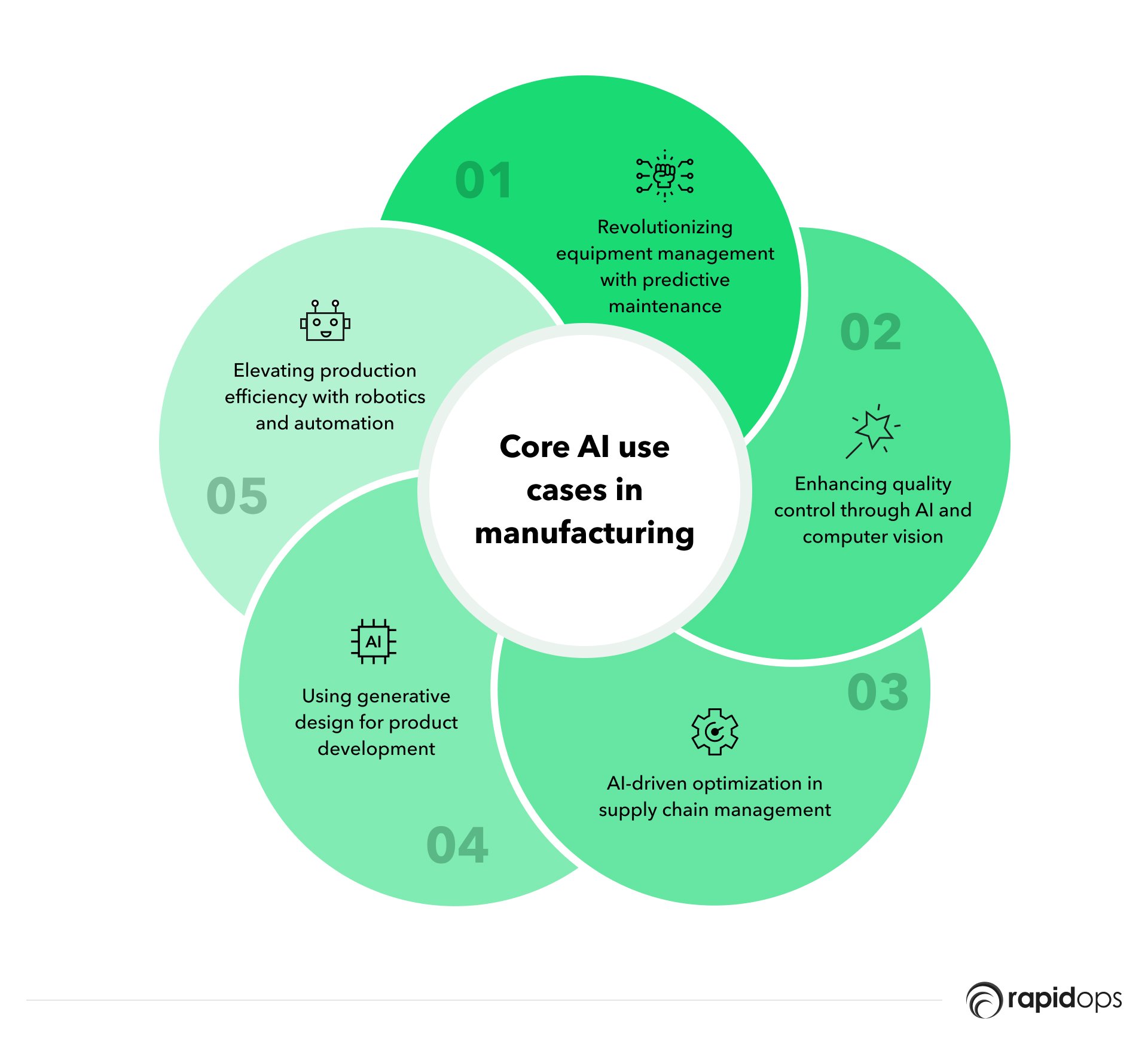
1. Predictive maintenance: Revolutionizing equipment management
Predictive maintenance represents a cornerstone AI application in manufacturing, drastically altering how manufacturers manage equipment maintenance.
By harnessing machine learning algorithms to analyze sensor data from machinery, AI systems can predict potential failures before they occur.
This predictive capability allows manufacturing plants to schedule maintenance proactively, significantly reducing unplanned downtime and maintenance costs.
Predictive maintenance not only exemplifies AI's impact on operational efficiency but also underscores its value in prolonging the lifespan of critical manufacturing assets.
2. Enhancing quality control through AI and computer vision
In the realm of quality control, artificial intelligence, particularly computer vision, has set new benchmarks for accuracy and efficiency.
Manufacturing facilities now deploy AI-powered robots and computer vision algorithms for real-time visual inspection of products on the assembly line.
These AI solutions offer exceptional precision in detecting defects, ensuring that products meet stringent quality standards.
The deployment of AI in quality control processes streamlines operations and significantly reduces the likelihood of costly recalls, safeguarding manufacturing companies' reputation and customer satisfaction.
3. Supply chain management: AI-driven optimization
AI technology has transformed supply chain management in the manufacturing industry, offering unprecedented insights for optimizing supply chains.
By analyzing vast datasets, including market trends and historical data, AI algorithms provide accurate demand forecasting, enabling manufacturing businesses to adjust their production processes and inventory management strategies accordingly.
This level of supply chain optimization not only ensures cost-effective operations but also enhances the agility and responsiveness of manufacturing companies to market demands.
4. Generative design: AI's role in product development
Generative design is an innovative AI use case where machine learning algorithms explore thousands of potential designs based on specified criteria such as materials, cost, and physical constraints.
This AI-based approach to product development allows for the rapid iteration and optimization of designs, enabling manufacturing companies to push the boundaries of innovation while reducing time-to-market for new products.
Generative design exemplifies how AI and machine learning contribute to the evolution of manufacturing processes, fostering creativity and efficiency in product development.
5. Robotics and automation: Elevating production efficiency
Integrating AI with robotics has ushered in a new manufacturing automation era.
AI-powered robots, equipped with advanced sensors and machine learning capabilities, work alongside human workers on the factory floor, enhancing production efficiency and safety.
These collaborative robots, or cobots, are designed to perform a wide range of tasks, from assembly to packaging, with precision and speed that surpass human capabilities.
AI in robotics optimizes the production line and introduces flexibility and adaptability to manufacturing processes, meeting the demands of modern manufacturing environments.
Check our infographic to know how AI is revolutionizing the manufacturing industry!
Download Now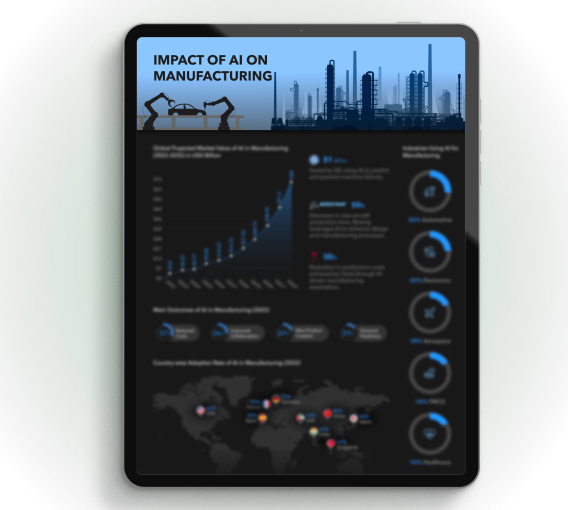
Impactful AI implementation stories in the manufacturing industry
Case Study 1: General Electric (GE) - Pioneering predictive maintenance

General Electric (GE) has been at the forefront of employing AI for predictive maintenance in its manufacturing operations.
By leveraging its Predix platform, GE analyzes data from sensors embedded in machinery to predict equipment failures before they happen.
This approach has led to significant reductions in unplanned downtime and maintenance costs across GE's manufacturing facilities, showcasing the potential of AI to transform traditional maintenance strategies into proactive, intelligence-driven actions.
Case Study 2: Intel - Enhancing quality control with AI
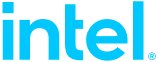
Intel, a global leader in the semiconductor industry, has implemented AI-driven solutions to improve quality control in its manufacturing processes.
Intel's AI algorithms use advanced computer vision systems to inspect silicon wafer fabrication and detect anomalies more precisely than human inspectors or traditional methods.
This AI-enhanced quality control process has not only improved the yield but also reduced the manufacturing lead time, demonstrating the impact of AI on ensuring product quality and operational efficiency.
Case Study 3: Siemens - Optimizing supply chains with AI
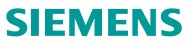
Siemens has effectively utilized AI to optimize its supply chain operations, particularly in its Digital Industries division.
By implementing AI-based analytics, Siemens can forecast demand more accurately, manage inventory levels efficiently, and enhance the overall agility of its supply chain.
This strategic use of AI has enabled Siemens to respond more swiftly to market changes, reduce excess inventory, and improve customer satisfaction by ensuring timely delivery of products.
Challenges of implementing AI in the manufacturing industry
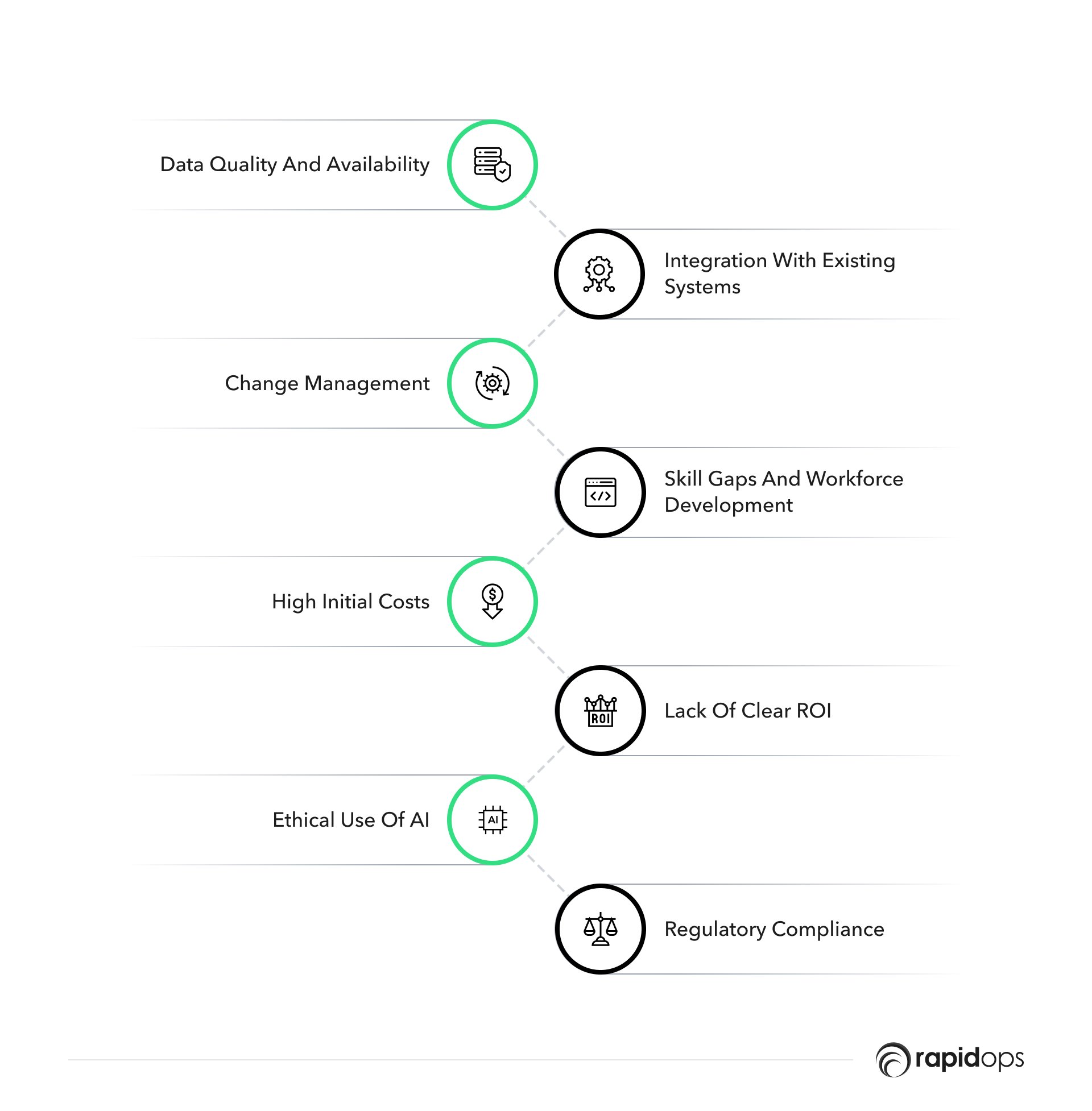
Implementing AI in the manufacturing industry presents various challenges, from technological hurdles to organizational and workforce-related issues.
Understanding these challenges is essential for developing effective strategies to overcome them and successfully leverage AI technologies.
Here are some key challenges along with potential solutions:
1. Data quality and availability
AI algorithms require large volumes of high-quality data to train and operate effectively. However, many manufacturing companies struggle with collecting, storing, and managing the necessary data due to legacy systems not designed for interoperability or real-time data capture.
Solution
Invest in upgrading data infrastructure to ensure compatibility with AI technologies and implement robust data governance practices to enhance data quality and availability.
2. Integration with existing systems
Integrating AI solutions with existing manufacturing systems and machinery can be complex, especially when dealing with outdated equipment or software.
Solution
Develop a phased integration plan that includes the modernization of legacy systems where feasible and the use of middleware or APIs to facilitate communication between old and new systems.
3. Change management
Adopting AI can significantly alter existing workflows, job roles, and organizational structures, potentially leading to resistance among employees who fear job displacement or lack the skills to work with new technologies.
Solution
Implement comprehensive change management strategies that include clear communication about the benefits of AI, retraining programs for employees, and involving workers in the transition process to alleviate fears and build buy-in.
4. Skill gaps and workforce development
The effective use of AI requires a workforce skilled in data science, machine learning, and AI application development. However, there is often a significant skill gap in the existing workforce.
Solution
Invest in training and development programs to upskill current employees and consider partnerships with educational institutions to ensure a steady pipeline of talent skilled in AI technologies.
5. High initial costs
The upfront investment required for AI technologies, including hardware, software, and talent acquisition, can be prohibitive for some manufacturers, particularly small and medium-sized enterprises (SMEs).
Solution
Explore cost-effective AI solutions, consider as-a-service models to reduce upfront costs, and seek government grants or incentives to support digital transformation in manufacturing.
6. Lack of clear ROI
Demonstrating the immediate return on investment (ROI) for AI projects can be challenging, as benefits may accrue over time and be difficult to quantify in the short term.
Solution
Set clear, measurable objectives for AI projects and use pilot programs to demonstrate potential ROI and operational improvements before committing to larger-scale implementations.
7. Ethical use of AI
Ensuring that AI is used ethically, particularly concerning data privacy, worker surveillance, and decision-making transparency, is a growing concern.
Solution
Develop ethical guidelines for AI use that prioritize transparency, fairness, and privacy and establish oversight mechanisms to monitor compliance.
8. Regulatory compliance
Navigating the complex landscape of regulations governing data use, AI applications, and automated decision-making can be challenging for manufacturers operating in multiple jurisdictions.
Solution
Stay informed about relevant regulations and standards and involve legal and compliance experts in developing and deploying AI solutions.
Overcoming these challenges requires a strategic approach that balances technological innovation with organizational readiness, workforce development, and ethical considerations.
By addressing these hurdles head-on, manufacturers can fully harness the transformative potential of AI in their operations.
Blueprint for AI integration in manufacturing
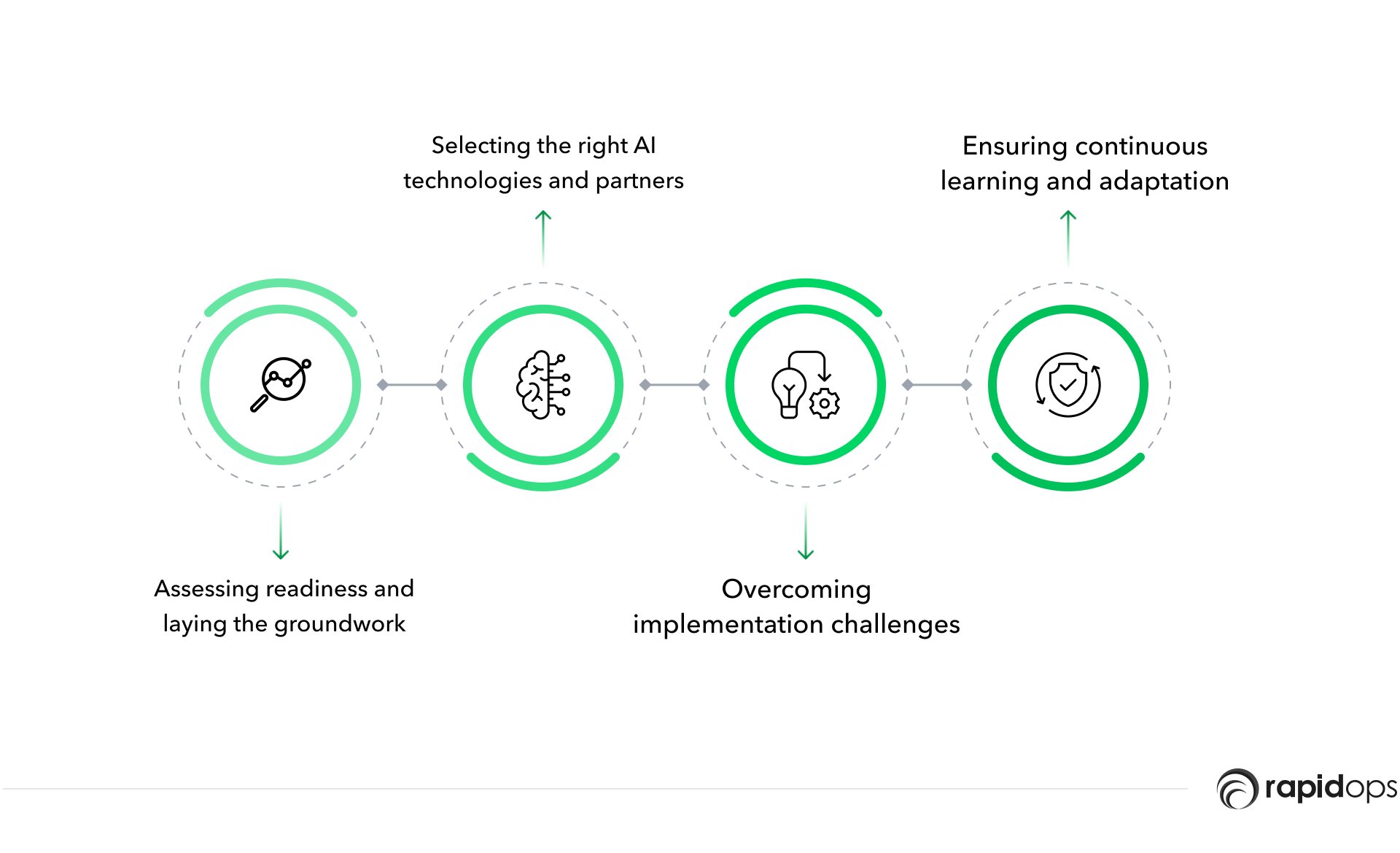
Assessing readiness and laying the groundwork
The first step towards integrating AI into manufacturing operations is assessing organizational readiness. This involves evaluating the current technological infrastructure, data management capabilities, and workforce skills.
Manufacturers must also identify areas where AI can deliver the most value, improving operational efficiency, enhancing product quality, or innovating supply chain management.
Establishing clear objectives and metrics for success early on helps align AI initiatives with business goals.
Selecting the right AI technologies and partners
Choosing the appropriate AI technologies and AI development company is crucial for modern manufacturers who want to tap into the potential artificial intelligence holds for faster scalability.
Manufacturers must focus on scalable, adaptable, and compatible solutions with their existing systems. It's equally important to select the right technology partners—companies with proven expertise in AI and a deep understanding of the manufacturing sector.
Collaborating with partners such as Rapidops, who can provide ongoing support while navigating the complexities of AI integration, will bear better fruits for their operational efficiency.
Overcoming implementation challenges
Implementing AI in manufacturing is not without its challenges. These can range from technical hurdles, such as data integration issues, to organizational challenges, like resistance to change.
Manufacturers can address these challenges by fostering a culture of innovation, providing comprehensive training for employees, and implementing pilot projects to demonstrate AI's potential benefits.
Pilot projects not only help in fine-tuning AI applications but also build confidence and buy-in across the organization.
Ensuring continuous learning and adaptation
The AI landscape is continually evolving, and so should AI strategies in manufacturing. This means regularly reviewing and updating AI initiatives to leverage new technologies and insights.
Encouraging a culture of continuous learning and experimentation is key. Manufacturers should also establish feedback loops that allow for the constant refinement of AI applications based on real-world performance and outcomes.
Concluding thoughts: A future-ready manufacturing process powered by artificial intelligence
The journey of embedding AI technologies into the fabric of manufacturing operations offers profound opportunities for transformation.
The imperative for business owners and decision-makers is clear:
- harness AI's potential to optimize current operations
- unlock new avenues for growth and innovation
This involves a financial investment in technology and a commitment to cultivating an organizational culture that embraces continuous learning, innovation, and adaptation.
As the manufacturing sector stands on the brink of a new digital era, adopting AI technologies emerges as a key differentiator. It offers a pathway to survive the complexities of today's market dynamics and thrive in them.
By leveraging AI, manufacturers can achieve operational excellence, enhance product quality, and drive innovation, setting a foundation for sustained success in an increasingly competitive and fast-paced world.
Here are some FAQs that delve into the realm of AI in manufacturing.
What are the primary benefits of implementing AI in manufacturing operations?
AI in manufacturing brings several key benefits, including increased operational efficiency, reduced production costs, improved product quality, and enhanced predictive maintenance capabilities. By leveraging AI, manufacturers can optimize their production processes, minimize waste, and anticipate equipment failures before they lead to costly downtimes.
How does AI enhance predictive maintenance in manufacturing plants?
AI enhances predictive maintenance by analyzing data from sensors embedded in machinery to detect patterns indicative of potential failures or wear and tear. By employing machine learning algorithms, these systems can predict when a machine is likely to fail or require maintenance, allowing for interventions to be scheduled at the most opportune times, thus minimizing disruption and extending the lifespan of equipment.
Can AI in manufacturing lead to job losses?
While AI automates certain tasks, it doesn't necessarily lead to job losses but rather shifts the types of roles required in the manufacturing sector. AI creates opportunities for higher-skilled jobs, such as AI system management, data analysis, and maintenance of advanced automated systems. Additionally, AI can relieve human workers from repetitive and hazardous tasks, reallocating human resources to more complex and creative roles, thereby enhancing job satisfaction and safety.
What role does AI play in manufacturing companies' supply chain and inventory management?
AI significantly improves supply chain and inventory management by providing real-time insights and predictive analytics. AI algorithms can forecast demand more accurately, optimize inventory levels, and identify the most efficient logistics routes. This reduces inventory costs and enhances the supply chain's responsiveness to market changes, improving customer satisfaction and operational agility.
How do manufacturers ensure the ethical use of AI in their operations?
Manufacturers can ensure the ethical use of AI by implementing transparent AI policies, prioritizing data privacy and security, and ensuring AI systems are designed to be fair and unbiased. This involves regular audits of AI systems for ethical considerations, employee training on the ethical use of AI, and engaging stakeholders in discussions about AI ethics to foster an environment of trust and responsibility.
How much does it cost to implement AI in manufacturing operations?
The cost of implementing AI in manufacturing operations can vary widely depending on several factors, including the scale of the implementation, the complexity of the AI solutions, the state of the existing infrastructure, and the specific goals of the AI initiatives. Here are key components that influence the overall cost:
- Initial Investment in Technology: This includes the cost of AI software and algorithms, hardware (if new equipment like sensors or upgraded machinery is required), and integration with existing systems. Depending on the solutions chosen, this can range from thousands to millions of dollars.
- Data Infrastructure: AI must have adequate data collection, storage, and management infrastructure. Costs may be incurred in upgrading data systems or migrating to cloud-based solutions to ensure AI systems have access to high-quality, real-time data.
- Talent Acquisition and Training: Hiring new employees with AI expertise or training existing staff to manage and operate AI systems is a significant part of the investment. This also includes ongoing education to keep up with evolving AI technologies.
- Maintenance and Upgrades: Continuous maintenance of AI systems, software updates, and possibly hardware upgrades contribute to the overall cost. This ensures that AI solutions remain effective and aligned with the latest technological advancements.
- Consultation and Partnership Fees: Working with AI consultants or entering partnerships with AI technology providers can accelerate the implementation process and add to the cost. These fees vary based on the scope of the project and the level of expertise required.
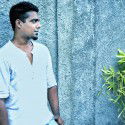
Saptarshi Das
Content Editor
9+ years of expertise in content marketing, SEO, and SERP research. Creates informative, engaging content to achieve marketing goals. Empathetic approach and deep understanding of target audience needs. Expert in SEO optimization for maximum visibility. Your ideal content marketing strategist.
What’s Inside
- AI in use cases in manufacturing: Key insights for business leaders
- The transformative impact of AI on manufacturing processes
- Core AI use cases in manufacturing
- Impactful AI implementation stories in the manufacturing industry
- Challenges of implementing AI in the manufacturing industry
- Blueprint for AI integration in manufacturing
- Concluding thoughts: A future-ready manufacturing process powered by artificial intelligence
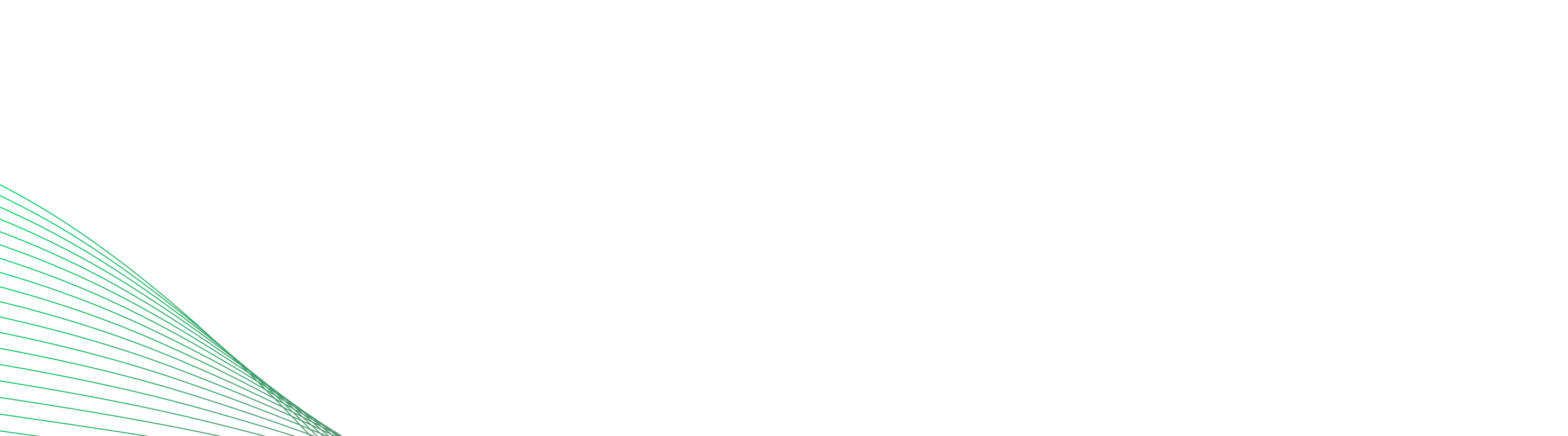
Let’s build the next big thing!
Share your ideas and vision with us to explore your digital opportunities
Similar Stories
- AI
- 4 Mins
- September 2022
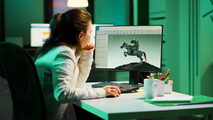
- AI
- 9 Mins
- January 2023
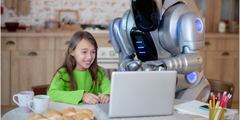
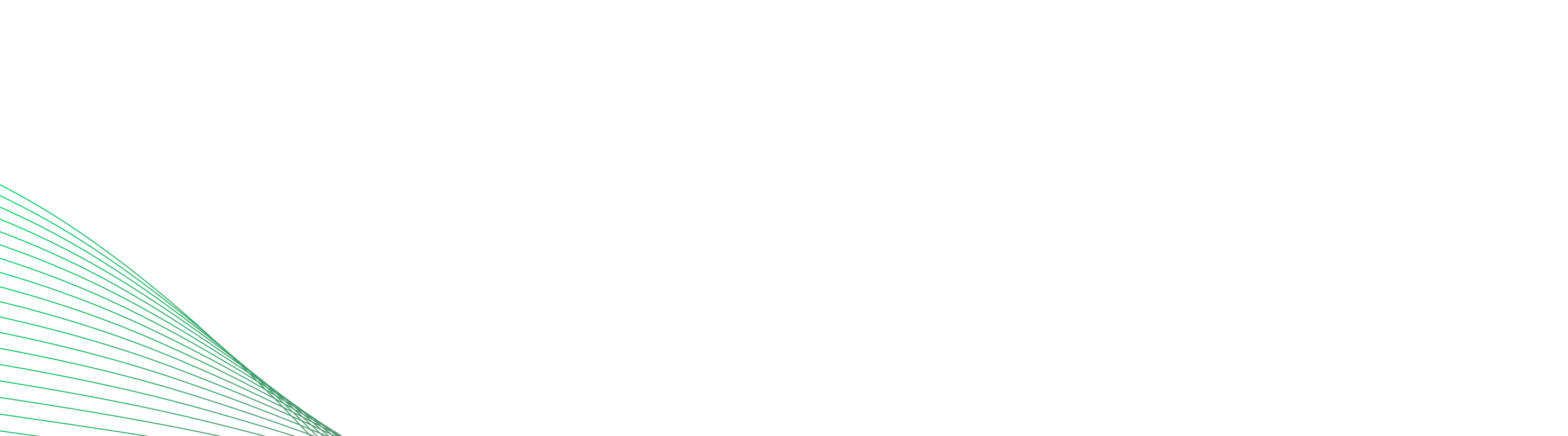
Receive articles like this in your mailbox
Sign up to get weekly insights & inspiration in your inbox.