Cyber threats are evolving. Is your defense ready to keep up?
Cybercriminals aren’t just evolving. They’re using AI to think, attack, and adapt faster than ever. In the past year alone, AI-powered cyberattacks surged by 300%, fueling ultra-realistic phishing scams, deepfake fraud, and malware that outsmart traditional defenses. Meanwhile, businesses are still relying on outdated, reactive security measures, leaving critical vulnerabilities exposed.
The consequences are staggering: It takes an average of 277 days to detect and contain a breach, costing companies $4.45 million per incident, not to mention irreparable damage to brand reputation. Yet, many businesses still wait to react to threats rather than proactively prevent them.
But what if your security could outthink and outpace attackers? This is where Generative AI is transforming cybersecurity. It doesn’t just detect threats. It predicts, adapts, and neutralizes them in real-time. AI-driven security identifies hidden vulnerabilities before attackers even notice them, generates adaptive threat responses, and automates remediation at machine speed.
In this article, we’ll explore seven powerful ways Generative AI is redefining cybersecurity, enabling businesses to stay ahead of cybercriminals, strengthen defenses, and reduce risks before they escalate into costly breaches.
1. AI-generated threat intelligence and predictive analysis
In today’s hyper-connected world, cyber threats evolve faster than ever before, outpacing traditional security defenses. Reactive cybersecurity strategies simply can’t keep up. Organizations need predictive intelligence to anticipate and neutralize threats before they materialize. This is where Generative AI steps in, revolutionizing the way we defend against cyber risks.
How generative AI enhances threat intelligence and predictive analysis
Real-time cyber threat detection
Generative AI autonomously analyzes vast datasets, network logs, dark web activity, and global cyber incidents, spotting threats in real time that human analysts may miss. By identifying patterns and anomalies, AI-generated threat reports provide actionable insights, allowing security teams to respond instantly, neutralizing potential risks before they can escalate.
Example: AI-driven threat intelligence detected a major phishing campaign in 2023, saving companies millions in damages by flagging it before it reached critical systems.
Predictive threat modeling
Generative AI doesn’t just react, it predicts. By simulating potential cyberattack scenarios using real-world data, AI enables proactive defense strategies.
Security teams can anticipate tactics cybercriminals may use, allowing them to implement countermeasures before an attack even happens. This predictive approach not only reduces response time but also minimizes financial and reputational damage.
Example: AI-driven threat models successfully helped a financial institution prepare for a potential zero-day attack, preventing a breach that could’ve compromised millions in customer data.
Autonomous threat hunting and anomaly detection
AI continuously scans network activity, detecting zero-day threats and sophisticated attack vectors in real-time. Unlike traditional, rule-based detection systems, Generative AI can identify unknown threats, providing organizations with a powerful defense against evolving cyber risks.
Automated AI-driven threat hunting reduces the need for manual intervention, allowing security experts to focus on higher-priority issues.
Example: Google’s AI-powered defense successfully blocked a sophisticated zero-day attack, showcasing the power of AI to identify complex threats before they infiltrate a system.
Automated threat intelligence reports
Generative AI doesn’t just stop at detection, it translates complex cybersecurity data into executive-level insights, helping C-suite leaders make fast, data-driven decisions. AI-powered reports provide predictive risk assessments, enabling businesses to prioritize cybersecurity investments and allocate resources effectively. Simulations of potential threats, such as ransomware attacks, data breaches, and insider threats—prepare executives to make proactive decisions.
Example: AI-powered simulations allowed a major corporation to prepare for a ransomware attack, ensuring its security infrastructure was fortified before the breach occurred.
2. Automated phishing detection and response
Phishing remains a major cybersecurity threat, with attacks growing by 35% in 2020 alone, leading to over $1.8 billion in losses. Traditional anti-phishing methods struggle to keep up with evolving tactics.
Generative AI transforms phishing detection by enabling real-time, adaptive security measures that proactively identify and neutralize threats. Unlike static rule-based systems, AI continuously learns and predicts new phishing patterns, offering a proactive defense to prevent financial and reputational damage before it occurs.
How generative AI powers phishing detection and response
Generative AI strengthens phishing defense by spotting patterns, understanding intent, and enabling faster, smarter responses.
AI-driven email and message analysis
Generative AI scans and analyzes emails, SMS, and social media messages in real time, identifying phishing patterns that could bypass traditional filters. By evaluating writing styles, embedded links, and metadata, AI distinguishes between legitimate communication and fraudulent attempts, ensuring rapid and accurate detection.
Example: A leading financial institution implemented Generative AI to analyze emails from external sources. The AI was able to detect a sophisticated phishing attempt that traditional email filters missed, preventing a potential $3 million loss.
Real-time threat simulation and training
Generative AI creates hyper-realistic phishing simulations, allowing organizations to train employees against advanced phishing tactics.
These AI-generated attack scenarios closely mirror real-world threats, enhancing employee awareness and preparedness. AI continuously updates the simulations, ensuring training stays relevant as phishing techniques evolve.
Risk factor: A recent study revealed that 91% of cyberattacks start with a phishing email, making employee training critical. Without real-time, AI-powered simulations, companies risk higher vulnerability due to employee mistakes.
Automated threat response and mitigation
Once a phishing attempt is detected, Generative AI acts immediately to isolate malicious emails, prevent them from reaching employees, and mitigate potential damage. It automatically disables compromised accounts, flags suspicious activities, and alerts security teams in real time, minimizing disruptions and enhancing overall security posture.
Example: A global eCommerce platform implemented generative AI for automated threat response. When a phishing email targeted an executive, the AI swiftly disabled the compromised account and alerted the security team, averting potential damage from a data breach.
Deepfake and impersonation detection
Generative AI analyzes voice, video, and text data to identify AI-generated phishing attacks, such as deepfake CEO fraud. These attacks exploit human trust by impersonating key executives or employees. By recognizing discrepancies in visual, audio, and textual data, AI helps companies combat these sophisticated social engineering tactics.
Competitor insight: Competitors in the cybersecurity space, such as Darktrace, have also integrated AI for deepfake and impersonation detection. However, their focus is predominantly on network monitoring, whereas Generative AI applies to a broader range of phishing and impersonation tactics, offering a more comprehensive defense strategy.
3. AI-powered anomaly detection for network security
Network security is facing increasing challenges with rapidly evolving cyber threats. Traditional systems often struggle to keep up with new attack methods, relying on known patterns to identify risks.
Generative AI is transforming network security by analyzing real-time activity, predicting vulnerabilities, and enabling proactive, adaptive defense strategies that go beyond traditional methods.
The rise in zero-day vulnerabilities and advanced persistent threats (APTs) means that businesses need more advanced solutions to protect their systems. A report from Symantec found that 71% of all cyberattacks are carried out using advanced techniques that evade traditional detection methods.
Generative AI provides the edge organizations need to stay ahead of these evolving threats.
How generative AI enhances anomaly detection and network security
Generative AI improves network security by learning patterns, detecting anomalies in real time, and simulating threats to predict attacks.
Predictive detection of unknown threats
Unlike traditional systems, which rely on a set of rules and known signatures, Generative AI can predict new attack patterns and identify zero-day vulnerabilities by analyzing network behavior. It creates models of normal versus abnormal activity, enabling it to anticipate threats before they manifest.
Risk Factor: The reliance on predefined signatures in traditional systems can leave companies vulnerable to zero-day attacks, which are often used to bypass standard defenses. Generative AI’s predictive capability significantly strengthens an organization's defense by identifying unknown threats before they can do damage.
Real-time threat detection and risk mitigation
Generative AI continuously monitors network traffic, identifying abnormal patterns such as unexpected data flows, unusual logins, and unauthorized access attempts. These anomalies could be early signs of cyber threats, enabling security teams to address them before they escalate into full-blown attacks.
Comparison to traditional detection: Traditional rule-based systems rely on predefined attack signatures and known patterns to detect threats. This approach is limited because it cannot anticipate new or previously unseen attack vectors. In contrast, Generative AI doesn’t depend on signatures. It can learn from patterns of legitimate activity and detect subtle, novel anomalies indicative of emerging threats.
Behavioral analysis & adaptive security
Generative AI uses advanced behavioral analytics to model typical user behavior, device interactions, and system activities. If a user or system deviates from these learned patterns, AI can flag it for further investigation, identifying potential internal threats, such as privileged account abuse, or external threats like botnet infections.
Example: Google uses AI-driven defense systems that analyze user behavior to detect unusual login activity or access from unauthorized devices, helping prevent credential theft or insider threats in real-time.
Continuous learning and evolution
One of the most significant advantages of generative AI is its self-learning capability. It continuously evolves based on new data, allowing it to detect increasingly sophisticated attacks over time. Unlike traditional systems, which require periodic updates to remain effective, AI-powered security can adapt to emerging threats without manual reconfiguration.
Example: As cyberattacks become more sophisticated, traditional systems often struggle to keep up. In contrast, AI-powered solutions continuously improve, learning from new attack vectors and adapting to emerging threats in real-time.
Reducing false positives
Generative AI’s ability to understand context and behavior ensures that it can filter out irrelevant anomalies and reduce false positives, which often overwhelm security teams. By fine-tuning its threat detection models, AI ensures that security teams focus only on real threats, improving efficiency and response times.
Competitor Insight: While competitors like Darktrace use AI for anomaly detection in network security, Generative AI stands out by offering a more comprehensive solution that not only detects new threats but also adapts to constantly evolving network activity. This results in fewer false positives and a more focused approach to threat mitigation.
4. AI-generated adaptive authentication and fraud prevention
Traditional authentication methods, such as static passwords and fixed verification steps, are increasingly vulnerable to evolving cyber threats. Fraudsters now employ AI-driven attacks, credential stuffing, and deepfake-powered social engineering to bypass these traditional security measures.
Generative AI transforms authentication and fraud prevention by making security dynamic, intelligent, and context-aware, providing more robust protection against these sophisticated attacks.
How generative AI enhances adaptive authentication and fraud prevention
Generative AI strengthens adaptive authentication by analyzing behavior patterns, detecting fraud in real time, and enabling dynamic, context-aware security responses.
Behavior-based adaptive authentication
Generative AI continuously analyzes user behavior patterns, such as typing speed, device usage, and location. When an activity deviates from these normal behaviors, the system automatically escalates authentication steps or blocks access, preventing unauthorized entry.
Example: Amazon employs AI-driven authentication in their eCommerce platform. When a user attempts to log in from an unusual location or device, the system prompts for additional verification, like biometric checks, effectively preventing fraudulent account access.
AI-powered risk scoring and access control
Traditional security systems use a blanket approach to authentication, requiring the same steps for all users. In contrast, Generative AI assigns real-time risk scores to each login attempt. Low-risk users experience frictionless access, while high-risk activities automatically trigger more stringent security measures like multi-factor authentication (MFA) or biometric verification.
Example: Netflix uses AI-based risk scoring to differentiate between legitimate logins and suspicious access patterns. For example, if a user logs in from a new device or location, the system may flag the session for additional checks based on the risk score.
Deepfake and synthetic identity fraud detection
Generative AI has the ability to detect anomalies in facial recognition, voice authentication, and document verification, identifying fraudulent activities powered by AI technologies like deepfakes. It analyzes micro-expressions, voice modulations, and image inconsistencies to distinguish between real users and AI-generated fakes.
Example: In 2020, UAE banks deployed AI-powered deepfake detection to prevent fraudsters from using deepfake technology to impersonate clients during voice and video-based customer service interactions. The AI system flagged discrepancies in the voice’s tone and facial micro-expressions, stopping the fraud before any damage occurred.
Real-time payment and transaction fraud prevention
Generative AI plays a critical role in identifying abnormal spending patterns and detecting fraud attempts before they happen. It continuously monitors transactions across geographies, accounts, and devices, flagging unauthorized payments and reducing financial risks.
Example: Mastercard leverages generative AI to monitor real-time transaction activity. The system analyzes every transaction for patterns that deviate from the norm, such as a sudden large purchase in a new location. When AI identifies suspicious behavior, it can instantly flag the transaction and request additional verification, preventing financial losses.
5. Generative AI for incident response
Cybersecurity teams face an overwhelming volume of threats, making it increasingly difficult to detect, prioritize, and neutralize attacks in real time. Traditional incident response methods often rely heavily on manual intervention, leading to:
- Delayed response times, which escalate financial and reputational risks.
- Limited threat visibility, making it harder to detect sophisticated, multi-stage attacks.
- High operational costs, as security teams struggle to manage growing cyber threats manually.
Organizations must adopt a proactive, AI-driven approach to accelerate threat response and minimize risks. Generative AI enables cybersecurity teams to stay ahead of adversaries, offering a powerful tool for faster and more efficient threat mitigation.
How generative AI transforms incident response
Generative AI transforms incident response by automating threat analysis, accelerating decision-making, and generating contextual responses to contain and resolve attacks swiftly.
AI-powered threat detection and analysis
Generative AI continuously monitors network traffic, logs, and user behavior to identify malicious activity. Unlike traditional detection systems, which rely on predefined attack signatures, AI can detect anomalies, unknown attack patterns, and emerging threats in real-time, before they escalate into full-blown breaches.
Example: Darktrace, an AI-driven cybersecurity firm, uses machine learning to continuously monitor network activity. Their Enterprise Immune System can detect novel attack patterns, even those never seen before, by establishing a baseline of "normal" behavior for each network and detecting deviations from that baseline.
Automated incident investigation
Generative AI autonomously analyzes cyber incidents by correlating data from multiple sources, identifying attack patterns, origins, and potential vulnerabilities. This significantly reduces investigation times and empowers security teams to respond faster and more effectively.
Example: IBM's QRadar SIEM platform uses AI to rapidly analyze security logs and alerts. By automating the correlation of security events, it shortens the time needed to determine the root cause of incidents, enabling faster mitigation and reducing reliance on human analysts.
AI-generated attack simulations
Generative AI creates realistic cyberattack simulations to test security defenses and identify weaknesses before real threats occur. These simulations help organizations proactively fine-tune their security strategies and identify gaps in their defenses that could be exploited by real attackers.
Example: Cymulate, a cybersecurity company, uses AI-driven attack simulations to test an organization's security posture. Their attack surface management system helps businesses proactively identify vulnerabilities by simulating real-world attack scenarios.
Autonomous threat containment and remediation
Generative AI can autonomously isolate compromised endpoints, disable malicious processes, and apply security patches in real time, without human intervention. This reduces the time to contain and neutralize threats, minimizing damage and preventing the spread of attacks.
Example: CrowdStrike, a leader in endpoint security, uses AI to autonomously respond to cyber threats in real-time. The platform isolates infected devices, halts malicious processes, and prevents lateral movement across the network, ensuring that the threat is contained before it can spread further.
Predictive AI for proactive defense
Generative AI forecasts potential attack vectors by analyzing threat intelligence data and security trends, helping organizations anticipate and block future cyber threats before they even occur. By predicting the tactics, techniques, and procedures (TTPs) of threat actors, AI enhances defense preparedness.
Example: FireEye uses predictive AI to help organizations understand and prepare for emerging threats. By analyzing threat intelligence feeds and historical attack data, FireEye’s Threat Intelligence platform can predict the next move of cyber adversaries, helping businesses proactively prepare their defenses.
6. Insider threat detection and behavioral risk analysis
Insider threats whether caused by malicious employees, compromised accounts, or accidental data leaks, represent some of the most difficult cybersecurity risks to detect. Traditional security measures primarily focus on external threats, often leaving organizations vulnerable to:
- Data Breaches and intellectual property theft caused by insider misuse.
- Compromised Credentials that allow attackers to bypass security controls undetected.
- Operational Disruptions due to unauthorized system access and privilege misuse.
A reactive approach is no longer sufficient. Organizations need AI-driven solutions to proactively identify and mitigate insider risks before they escalate.
How generative AI strengthens insider threat detection and risk analysis
Generative AI strengthens insider threat detection by analyzing behavior patterns, identifying anomalies, and assessing risks with real-time, adaptive intelligence.
AI-powered behavioral analysis
Generative AI continuously learns from user behavior, identifying deviations that may signal potential threats. By analyzing patterns in login times, device usage, and data access, AI can detect unusual activities such as unauthorized logins or abnormal working hours that might go unnoticed with traditional methods.
Example: Microsoft’s Azure Sentinel leverages AI and machine learning to detect insider threats by continuously monitoring and analyzing user behavior.
Their UEBA (User and Entity Behavior Analytics) tool creates baseline behavior profiles for all users and flags deviations that could signal potential risks, like an employee downloading sensitive files outside of their usual working hours.
Advanced anomaly detection
Generative AI cross-references employee activities with historical data to uncover suspicious actions that traditional rule-based systems might overlook. For example, AI can spot unauthorized file transfers, access to restricted systems, or activities outside of typical working hours, all of which can be signs of malicious intent or compromised accounts.
Example: Varonis, a leading cybersecurity firm, uses AI-powered anomaly detection to monitor employee behavior across multiple data sources. Their Data Security Platform analyzes access patterns and flags anomalies, such as an employee accessing files they typically don't interact with, which could indicate a compromised account or malicious intent.
Predictive risk scoring
Generative AI assigns dynamic risk scores to users based on their behavioral trends, helping security teams prioritize potential threats before they escalate. These AI-generated risk scores assess factors such as unusual access patterns, increased activity levels, or high-risk transactions, providing real-time insights into potential insider threats.
Example: Splunk’s Phantom platform uses predictive analytics to assign dynamic risk scores to employees based on their activity within the system. These scores help organizations identify risky individuals and make informed decisions about when to intervene, reducing the potential for insider breaches.
Generative AI for insider threat simulations
Generative AI generates realistic insider threat scenarios to train security teams, helping them better understand and respond to internal risks. These AI-driven simulations expose vulnerabilities in security protocols, allowing organizations to refine their incident response strategies and improve their preparedness.
Example: Darktrace uses AI-generated threat simulations to test an organization's defense mechanisms. The platform creates real-world scenarios, including insider threats, and simulates them in a controlled environment, giving teams the opportunity to practice their response in real-time and improve their resilience against internal risks.
7. AI-driven malware and ransomware defense
Malware and ransomware attacks have evolved into sophisticated threats that can cripple businesses, disrupt operations, and lead to devastating financial losses. Traditional signature-based detection methods are becoming increasingly ineffective against rapidly mutating malware strains.
Generative AI transforms malware and ransomware defense by proactively identifying, analyzing, and neutralizing threats in real time, before they can infiltrate critical systems.
How generative AI powers malware and ransomware defense
Generative AI powers malware and ransomware defense by detecting threats early, simulating attack patterns, and enabling faster, automated threat containment.
AI-generated threat intelligence & predictive analysis
Generative AI continuously scans vast data sources, analyzing emerging ransomware patterns and predicting new malware variants before they spread. By leveraging machine learning and advanced threat intelligence models, AI strengthens defenses against zero-day attacks.
Example: Google’s AI-driven security systems detected and neutralized a new strain of ransomware within hours, preventing a large-scale breach.
Automated threat containment and response
AI-driven security frameworks automatically isolate infected systems, restrict access to critical files, and neutralize threats. By proactively preventing the spread of malware across networks, AI minimizes downtime and business disruption.
An example of this in action is when Microsoft's AI security system identified a ransomware outbreak and initiated automated containment, containing the attack within minutes and mitigating potential losses.
Deepfake and polymorphic malware identification
As cybercriminals increasingly use AI to create self-mutating malware that evades detection, Generative AI combats this by reverse-engineering polymorphic malware. By identifying attack patterns, AI neutralizes these sophisticated threats before they can execute, offering businesses a much-needed layer of protection against ever-evolving threats.
For instance, Symantec’s use of AI to combat polymorphic malware has demonstrated a significant increase in the detection rate of new malware strains, reducing exposure to ransomware attacks.
AI in cybersecurity: The right move at the right time
Cyber threats are evolving faster than ever, and traditional security models are struggling to keep up. Today, businesses need not just stronger defenses but smarter, more adaptive security strategies that evolve with the threats they face. Generative AI is already transforming cybersecurity by enhancing threat detection, preventing fraud, and automating incident response.
But for many leaders, the challenge isn’t just adopting AI, it’s understanding where and how it can drive real impact without disrupting operations. The key is starting with the right entry point. Where is your business most vulnerable? Could AI-driven automation help your team focus on high-priority threats instead of chasing false alarms?
Cyber threats won’t wait. Neither should you. AI-powered cyberattacks are evolving, is your defense adapting fast enough? The right AI-driven security strategy could be the difference between preventing a breach and becoming the next headline.
Let’s discuss how AI can fortify your cybersecurity, before attackers make their next move. Book a strategy call today.
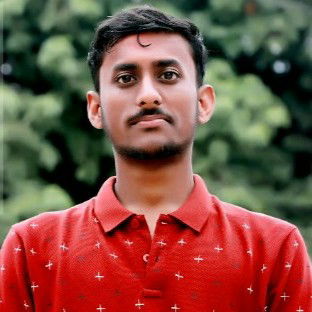
Rahul Chaudhary
Content Writer
With 5 years of experience in AI, software, and digital transformation, I’m passionate about making complex concepts easy to understand and apply. I create content that speaks to business leaders, offering practical, data-driven solutions that help you tackle real challenges and make informed decisions that drive growth.
What’s Inside
- 1. AI-generated threat intelligence and predictive analysis
- 2. Automated phishing detection and response
- 3. AI-powered anomaly detection for network security
- 4. AI-generated adaptive authentication and fraud prevention
- 5. Generative AI for incident response
- 6. Insider threat detection and behavioral risk analysis
- 7. AI-driven malware and ransomware defense
- AI in cybersecurity: The right move at the right time
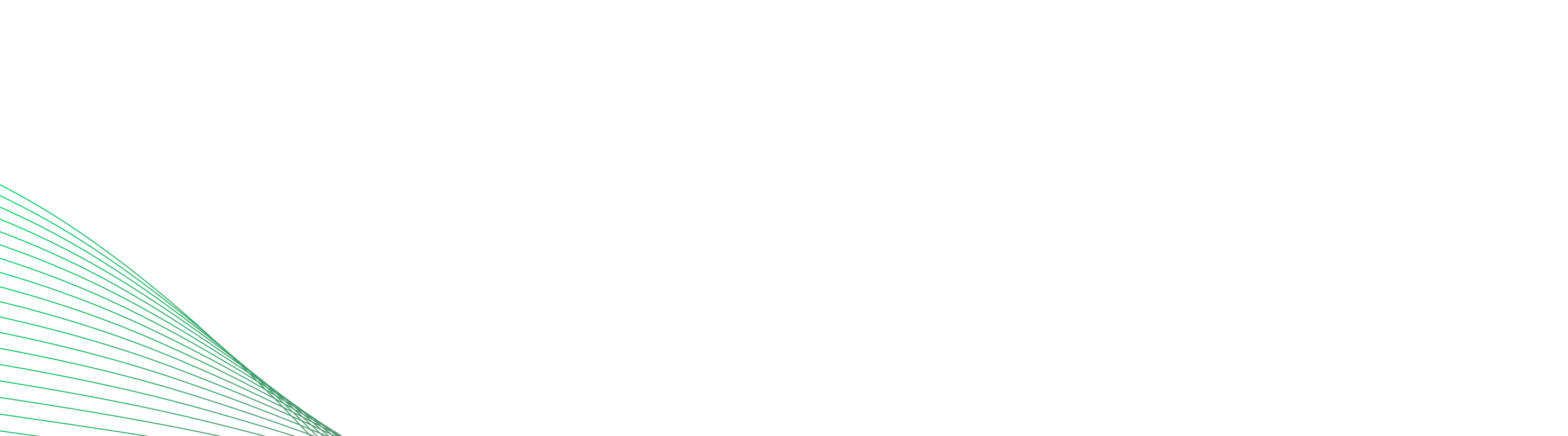
Let’s build the next big thing!
Share your ideas and vision with us to explore your digital opportunities
Similar Stories
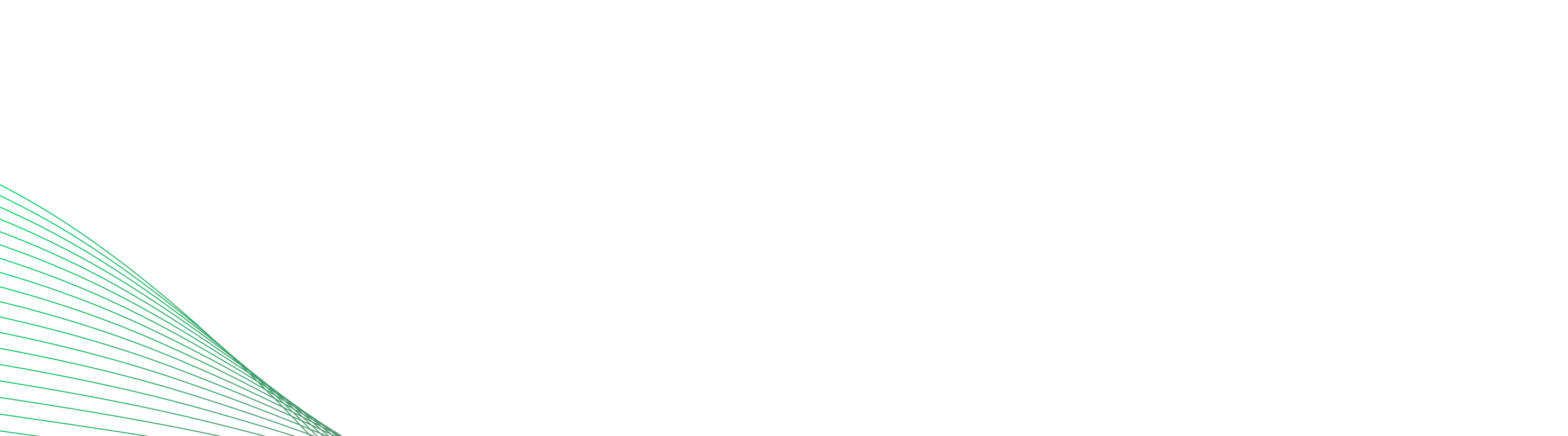
Receive articles like this in your mailbox
Sign up to get weekly insights & inspiration in your inbox.