- Analytics
- 7 min read
- March 2023
Supercharge Your Operational Efficiency with Data Analytics
By 2025, 463 exabytes of data will be created each day. - Forbes
It's no secret that data analytics provides valuable insights into a business's operations, customers, and market trends. Companies that successfully leverage it have been able to make more informed decisions that lead to:
- Increased efficiency
- Reduced costs
- Improved productivity
- Better customer experiences
But with stronger competition entering the market, businesses that do not embrace data analytics are at risk of falling behind. Integrating data analytics into business strategy has become critical for growth and success, especially when it comes to operational efficiency.
Data analytics can uncover inefficiencies within an organization's operations that might go unnoticed. By scrutinizing massive volumes of data from different sources, businesses can spot trends and patterns that signal opportunities to streamline their processes.
As a result, companies can save on costs, and gain helpful information about their customers, products, and services, allowing them to stay ahead of the competition.
In this article, we'll cover how you can harness the power of data analytics to become more efficient, gain a competitive edge, and take your business to the next level. Let's dive in.
Why does your business need data analytics?
Data and analytics are crucial in enhancing operational efficiency in different industries. In manufacturing, data from various sources such as sensors and IoT devices can be analyzed to optimize production processes, reduce downtime, and increase productivity.
Predictive maintenance can be used to avoid unplanned downtime and reduce maintenance costs, while optimizing inventory levels can reduce waste and enhance supply chain efficiency.
As per a PwC report, predictive maintenance in manufacturing could:
- Improve uptime by 9%
- Reduce costs by 12%
- Reduce safety, health, environmental & quality risks by 14%
- Extend the lifetime of aging assets by 20%
Another way that data and analytics can improve operational efficiency is by optimizing inventory levels. Retailers and manufacturers that use data analytics to optimize their supply chains can reduce inventory levels by up to 30%.
By analyzing historical sales data, manufacturers can better forecast demand and adjust inventory levels, reducing waste and increasing efficiency.
Data and analytics can also help manufacturers improve their overall equipment effectiveness (OEE), a measure of how effectively a machine is being used.
By analyzing data from sensors and other sources, manufacturers can identify bottlenecks and areas for improvement, leading to higher OEE and increased efficiency.
According to survey data from LNS Research, manufacturers who have real-time visibility into EHS metrics experience a significant 21% improvement in their Overall Equipment Effectiveness (OEE), outperforming those who lack such visibility.
How can businesses utilize data to become more efficient?
Expanding a business is a monumental task that requires exceptional foresight, dedication, and skill. The stakes are even higher if you've built your business from scratch.
However, the path to success lies at the intersection of solid business understanding and hard work.
To truly understand the needs of your ideal customers, their location, and how to reach them, you must collect and effectively use the data that drives, informs, and motivates action.
This is where data analytics with proper data governance, predictive capabilities, and planning functionality can make a significant difference.
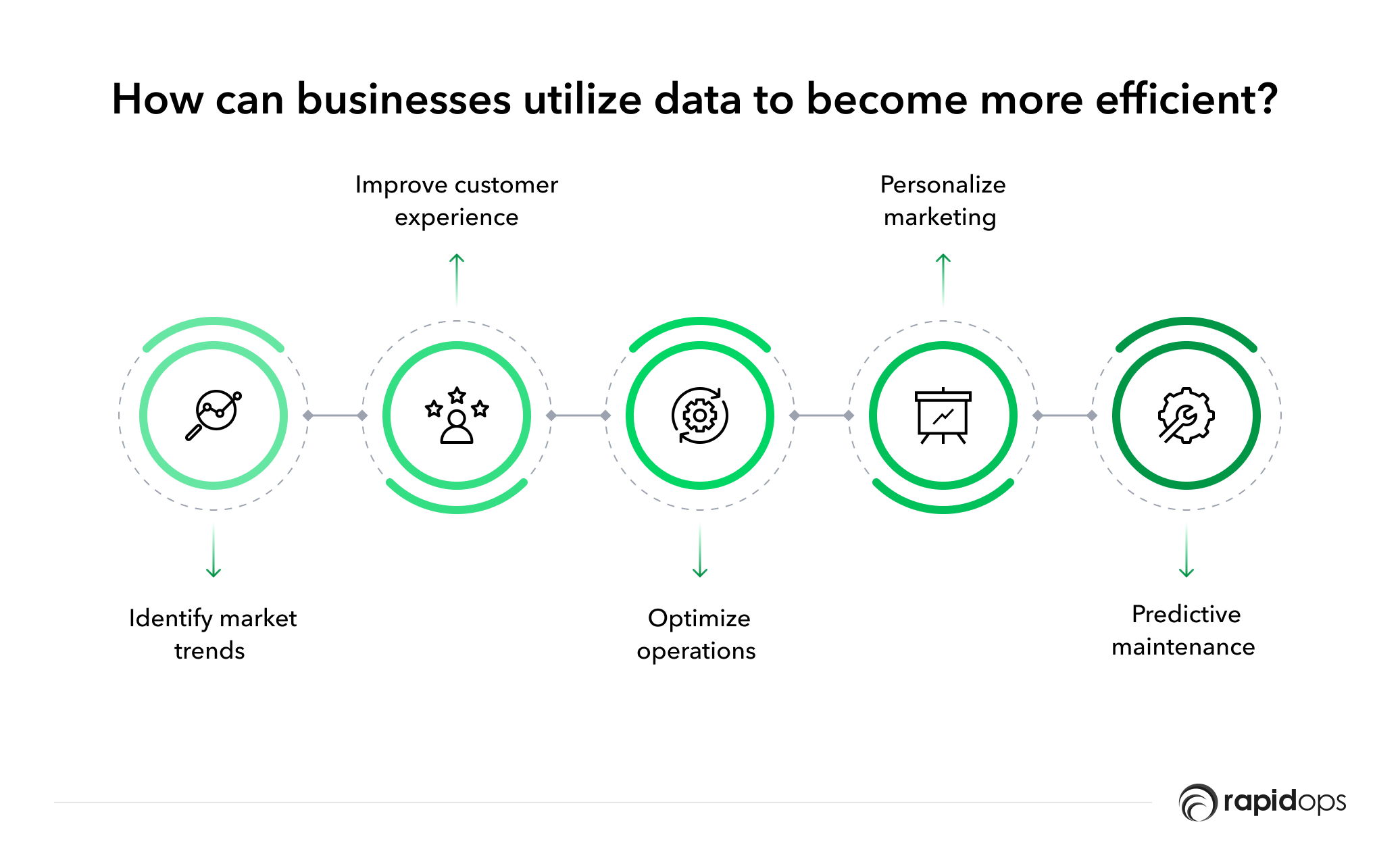
1. Identify market trends
Data analytics lets you gather insights into consumer behavior, preferences, and trends. This information can help you identify emerging market trends and predict future demand for your products or services.
Armed with this knowledge, you can make informed business decisions such as developing new products, expanding to new markets, or adjusting pricing strategies to stay competitive.
2. Improve customer experience
Analyzing customer data can help you better understand their needs and pain points throughout their journey with your business. This includes identifying areas for improvement in customer service, product design, or delivery processes.
Optimizing these areas can improve customer satisfaction, build brand loyalty, and increase customer lifetime value.
3. Optimize operations
Data analytics can help you identify inefficiencies in your business processes and operations.
For example, you can analyze your supply chain to identify bottlenecks or optimize inventory management to reduce costs. Optimizing these processes can reduce costs, improve productivity, and increase profitability.
4. Personalize marketing
With data analytics, you can segment your customers based on their behavior, preferences, and demographics.
This allows you to create personalized marketing messages and offers that resonate with each customer segment. Personalized marketing can increase engagement, conversions, and customer loyalty.
5. Predictive maintenance
Data analytics can help you predict when maintenance is needed for equipment or machinery. This can prevent costly downtime, extend the life of your equipment, and reduce maintenance costs.
By proactively addressing maintenance needs, you can also improve safety and reduce the risk of accidents.
[data_analytics_essentials image="https://www.rapidops.com/blog/wp-content/uploads/2023/03/Cover-Banner-updated-2.png" button_url="https://www.rapidops.com/resources/data-analytics-essentials/"]
How can better data quality help with efficient data analytics?
Poor data quality annually costs organizations an average of $12.9 million. - Source
Before businesses or decision-makers implement data analytics in their business plan, data quality is the primary factor to mind here.
High-quality data is crucial in gaining better customer insights, generating higher-quality leads, and fostering more robust customer relationships.
To gain a competitive advantage, leaders in data and analytics must continually strive to improve data quality. Let's understand how businesses can achieve successful data quality enhancements.
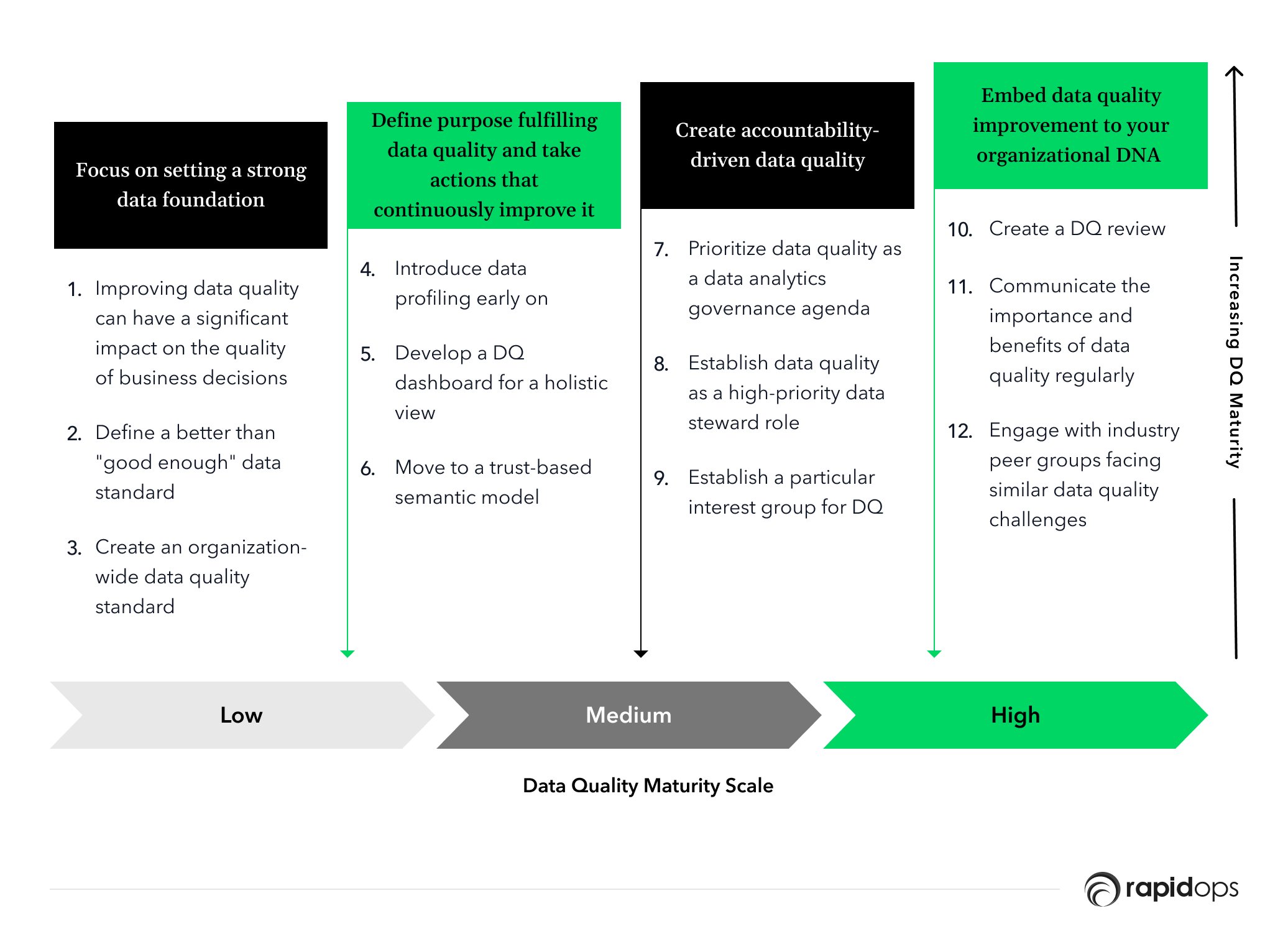
Focus on setting a strong data foundation
1. Improving data quality can have a significant impact on the quality of business decisions
When data is of high quality, it enables decision-makers to understand their customers and business operations better. Leverage this understanding to supercharge processes, elevate customer satisfaction, and skyrocket revenue.
To establish the connection between data quality and business decisions, it is essential to identify the existing data quality issues the organization faces and how they impact revenue and other business KPIs.
This requires a clear linkage between business processes, KPIs, and data assets.
With the connection established, data and analytics gurus craft laser-focused data quality improvement programs. This program should define the scope of the effort, the stakeholders involved, and a high-level investment plan.
By continuously improving data quality, businesses can gain a competitive advantage, improve their decision-making capabilities, and ultimately drive growth and success.
2. Define a better than "good enough" data standard
Defining a "good enough" standard of data requires understanding the specific needs and expectations of the organization.
It's the responsibility of the business to determine what level of data quality is necessary for their operations, and D&A leaders should have open communication with business stakeholders to capture their expectations.
For example, different lines of business may have different standards for the same type of data, such as customer master data.
By understanding these varying needs, D&A leaders can develop a data quality improvement program that aligns with the organization's overall goals and priorities.
3. Create an organization-wide data quality standard
Data and analytics (D&A) leaders can begin by identifying the critical data elements that require standardization and defining the rules around them.
Communicate these rules throughout the organization, ensuring all units and stakeholders embrace and adhere to the same high standards.
The DQ standard should also address the frequency of data checks, validation processes, and methods for resolving data quality issues.
This will help to create a culture of data quality across the organization, where everyone understands the importance of accurate data and takes responsibility for maintaining it.
The D&A leaders should periodically review and update the DQ standard to ensure it remains relevant and practical.
This commitment fortifies ongoing data quality enhancement and empowers the organization to make insightful business decisions based on rock-solid data.
Define purpose fulfilling data quality and take actions that continuously improve it
4. Introduce data profiling early on
Data profiling means examining data from an existing source and summarizing information about the data.
It uncovers corrective actions, offers invaluable insights for improvement plans, and pinpoints which data quality issues demand immediate attention.
Perform data profiling as often as feasible to maximize benefits.
For example, profiling could reveal that some critical customer contact information is missing. This missing information may have directly contributed to a high volume of customer complaints and would make good customer service difficult.
DQ improvement in this context has now become a high-priority activity.
5. Develop a DQ dashboard for a holistic view
Designing and implementing DQ dashboards can benefit the organization by providing all stakeholders with a holistic view of data quality.
Customizable DQ dashboards keep a watchful eye on crucial data assets, revealing trends and patterns for designing process enhancements and reflecting the impact of improvement efforts.
This enables the organization to make informed decisions and achieve its desired business objectives based on trusted quality data.
6. Move to a trust-based semantic model
Embracing a trust-based semantic model acknowledges the reality of unpredictable data quality, particularly from external sources.
Organizations must consider the source of data, its level of governance, and its accuracy. This means that organizations must implement mitigation measures to minimize the risks associated with using data that is not 100% trustworthy.
This can include establishing trust frameworks, performing regular data quality assessments, and implementing data governance policies and procedures.
By using a trust-based semantic model, organizations can ensure that they make informed decisions based on reliable data.
Create accountability-driven data quality
7. Prioritize data quality as a data analytics governance agenda
Prioritizing DQ as a regular agenda item at D&A governance board meetings is crucial to ensuring that data quality remains a top priority for the organization.
By linking DQ initiatives to business outcomes and monitoring progress against objectives, you guarantee alignment and accountability.
By regularly reviewing and discussing DQ improvements, the board can make informed decisions regarding resource allocation and ensure that the organization is on track to meet its goals.
Identifying challenges and roadblocks along the way fuels the momentum of the DQ improvement program and paves the way for success.
8. Establish data quality as a high-priority data steward role
By incorporating DQ responsibilities and procedures into the data steward role, organizations effectively manage and maintain data.
Defining data steward roles, establishing monitoring and reporting processes, and providing training and support guarantees timely resolution of data quality issues and efficient utilization of data assets for organizational success.
9. Establish a particular interest group for DQ
Establishing a particular interest group for DQ across BUs and IT is a great way to improve DQ. The chief data officer team or an equivalent body can lead this group.
Through joint DQ improvement efforts, the group systematically measures and maintains exceptional data quality.
This group can also help identify and address issues impacting data quality across the organization.
By working together, the group can remember best practices for improving data quality and share these practices across the organization. This can help to reduce operational costs and improve business outcomes.
In addition, this group can help to improve risk management by identifying and addressing issues that could impact the organization's data quality.
United as a group, they safeguard high-level data quality and promote effective data utilization to achieve organizational goals and objectives.
Embed data quality improvement to your organizational DNA
10. Create a DQ review
Implementing a DQ review as a "stage gate" in release management guarantees data quality verification before new assets enter production, preventing poor-quality data issues and ensuring optimal data usage in business processes.
By making DQ reviews a part of the release management process, D&A leaders can ensure that DQ is a critical component of the organization's overall data management strategy.
Designing the review process to catch issues and track progress enables swift corrections and ongoing data quality enhancement.
As the organization's maturity in handling DQ initiatives improves, it's essential to identify and circulate best practices that have been impactful.
This knowledge-sharing can lead to more efficient and effective DQ practices and a better understanding of the benefits of improved data quality throughout the organization.
11. Communicate the importance and benefits of data quality regularly
In addition to communicating the benefits to the board, it's also important to regularly communicate the benefits of better DQ to the business departments.
This will help them understand the value of their contribution to DQ improvement and motivate them to continue prioritizing DQ in their work.
D&A leaders can use a variety of communication channels, such as newsletters, town hall meetings, and training sessions, to keep business departments informed of the progress and impact of DQ initiatives.
By doing so, they can help create a culture of data-driven decision-making and continuous improvement.
12. Engage with industry peer groups facing similar data quality challenges
Engaging with external/industry peer groups can provide valuable opportunities for data and analytics leaders to exchange knowledge and insights with other organizations facing similar challenges in data quality.
User groups from vendors and service providers can be a great way to connect with peers and learn about best practices and new trends.
Additionally, attending industry events and conferences can provide exposure to new ideas and innovations in the field. Maintaining a continuous learning mindset and staying updated with the latest DQ (data quality) developments is vital to continuously improving and driving business outcomes.
Concluding thoughts
In today's data-driven landscape, companies possess an immense amount of valuable data and cutting-edge technologies to harness its power.
However, time is a scarce resource, and businesses can't afford to waste it in analyzing data manually. That's where Rapidops' team of AI and data analytics experts come into play.
With our end-to-end data analytics services, we have helped enterprises make informed decisions by unlocking insights from data and leveraging them to enhance their efficiency, productivity, and profitability.
We also provide customized solutions tailored to each client's unique transformative needs and organizational goals so they can estimate value from their data at lightning speed.