- Analytics
- 10 min read
- July 2023
Fundamentals of Advanced Analytics: A Comprehensive Guide
In the era of data-driven decision-making, understanding the basics of advanced analytics is crucial for any business. Advanced analytics is a data analysis approach that employs predictive modeling, machine learning algorithms, deep learning, business process automation, and other statistical techniques to examine business information from various data sources.
Beyond traditional business intelligence (BI) methods, advanced analytics utilizes data science to identify patterns and estimate the likelihood of future events. This enables organizations to be more responsive and significantly improve the accuracy of their decision-making processes.
Importance of advanced analytics in business
Advanced analytics is invaluable to enterprises as it allows them to extract more functionality from their data assets, regardless of the data's location or format. It also helps address complex business issues that traditional BI reporting cannot tackle.
For instance, a consumer-packaged goods manufacturer aiming to develop a contextual marketing engine might need to answer the following questions:
- When is a customer likely to run out of a particular item?
- What time of day or week are they most receptive to marketing advertisements?
- What is the achievable profitability when marketing at that specific time?
- At what price point are they most likely to make a purchase?
By integrating consumption models with historical data and artificial intelligence (AI), advanced analytics can assist organizations in determining precise answers to these questions, allowing them to make informed decisions tailored to their business goals.
Benefits of advanced analytics in business
The integration of advanced analytics into business operations can unleash a myriad of benefits. Through sophisticated methodologies and data interpretation, businesses can enhance their operations and increase their understanding of consumer behavior. Here are some of the key benefits of advanced analytics in business:
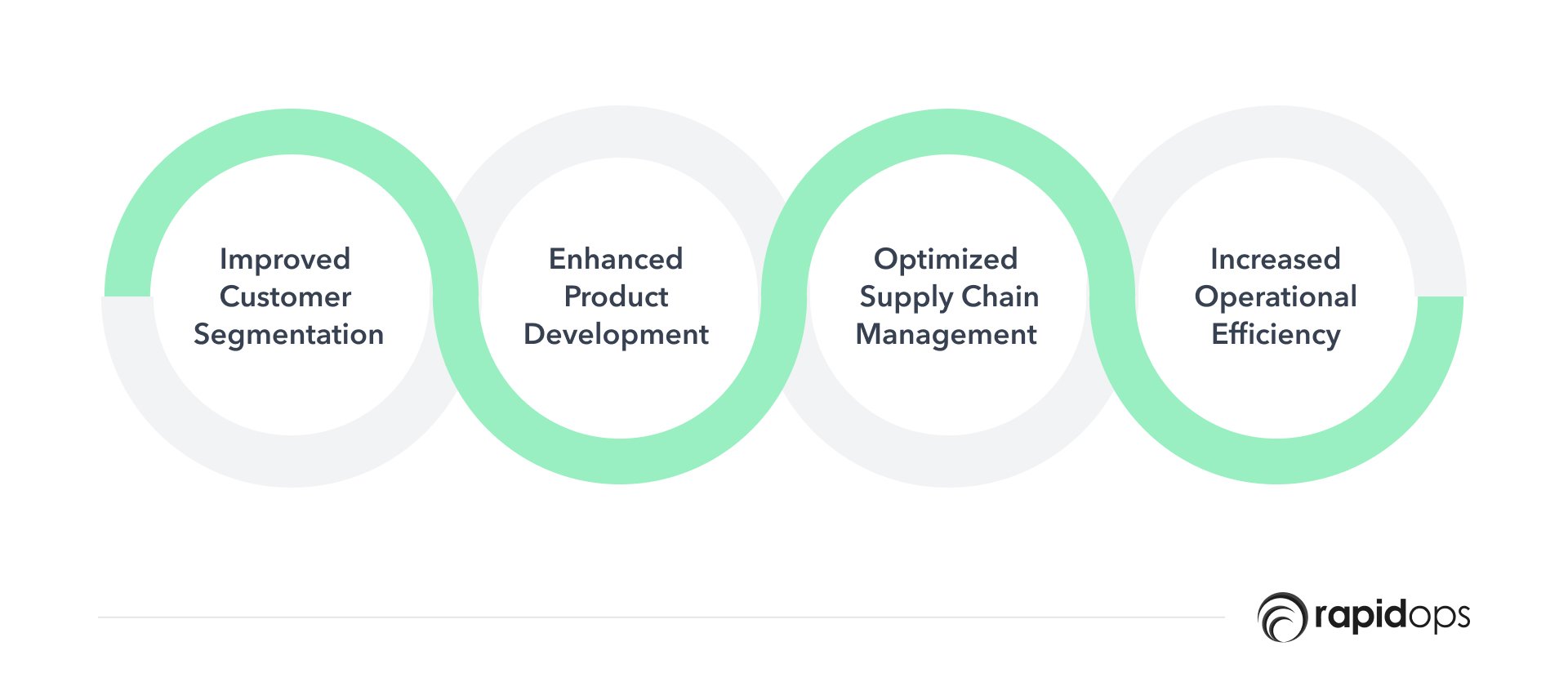
1. Improved customer segmentation
Advanced techniques such as cluster analysis and machine learning equip businesses with the ability to dissect their customer base more efficiently. This results in creating targeted marketing strategies, leading to higher engagement and conversion rates.
2. Enhanced product development
Conjoint analysis and perceptual mapping are pivotal in identifying customer preferences. Utilizing these insights, businesses can develop products that cater to specific needs, fostering customer satisfaction and loyalty.
3. Optimized supply chain management
Employing advanced analytics techniques like data mining and machine learning can drastically improve supply chain management. These techniques enable businesses to optimize their supply chains, reducing costs and bolstering efficiency.
4. Increased operational efficiency
By leveraging advanced techniques such as regression analysis and factor analysis, businesses can identify potential areas of improvement within their operations. This proactive approach to problem-solving often leads to the optimization of operations and significant enhancements in overall productivity.
Use cases of advanced analytics in business.
Advanced analytics have found their way into numerous aspects of the business world, proving invaluable in enhancing operations, reducing costs, and driving strategic decision-making. Let's delve into some practical examples of how businesses can wield the power of advanced data analytics:
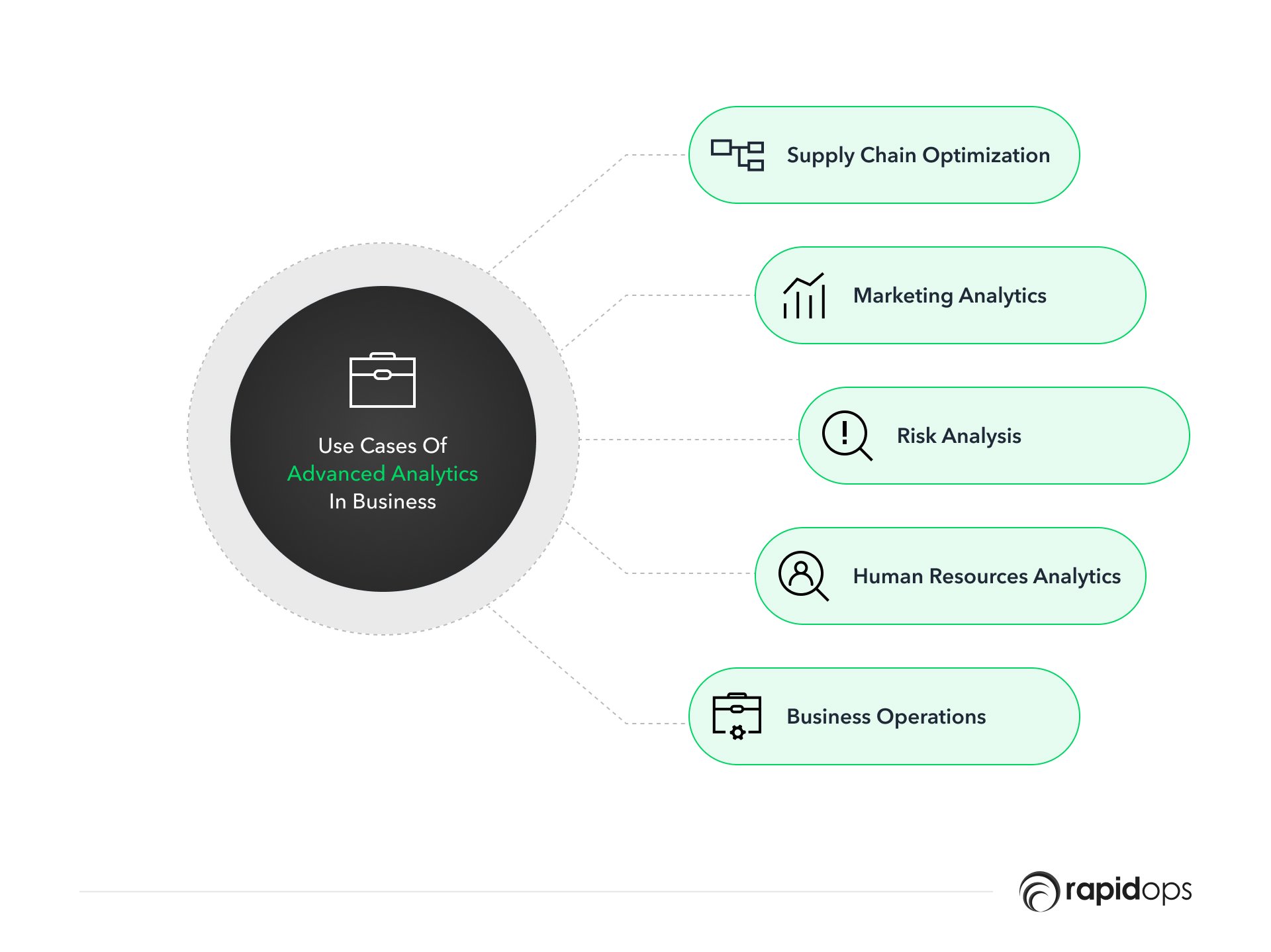
1. Supply chain optimization
For global enterprises, a responsive supply chain is crucial to adapt to changing market conditions, such as fluctuations in demand, customer preferences, and costs. Advanced analytics can be particularly helpful in manufacturing to minimize distribution expenses, prevent delays or equipment failures, and streamline processes.
For instance, a multinational electronics company could use advanced analytics to predict potential bottlenecks in its supply chain, allowing for timely adjustments and reducing downtime.
2. Marketing analytics
Gaining an understanding of customers is increasingly challenging, and delivering targeted marketing at the right time can be a daunting task. Advanced analytics enables companies to develop personalized sales and marketing campaigns, reduce expenditures on ineffective strategies, identify up-sell and cross-sell opportunities, and enhance customer experiences.
For example, a retail company could use advanced analytics to analyze customer purchase data and social media activity, tailoring marketing efforts to individual preferences and needs.
3. Risk analysis
Businesses aim to mitigate future risks, but only those utilizing advanced analytics can manage large data sets or real-time data streams to identify risk patterns. Advanced analytics techniques can detect fraudulent activities in payments and orders or monitor reputation among existing customers.
A financial institution, for example, could apply advanced analytics to identify suspicious transactions and prevent potential fraud.
4. Human resources analytics
Employee satisfaction significantly impacts business performance, but many companies overlook the value of human resource data. Advanced analytics can be employed in HR to lower employee turnover, reduce recruiting and hiring costs, and invest in effective rewards programs to maintain or increase employee satisfaction.
A large corporation could use advanced analytics to identify factors affecting employee engagement and develop targeted initiatives to improve morale and retention.
5. Business operations
Traditional BI tools often lack the agility needed for operational excellence that responds to changing market conditions and optimizes decisions based on future trend predictions. Advanced analytics enables organizations to enhance performance and productivity, increase revenue, and resolve operational issues more rapidly.
A manufacturing company could use advanced analytics to optimize production schedules, streamline inventory management, and identify potential process improvements, ultimately boosting overall efficiency.
Advanced data analytics techniques
In the modern business landscape, advanced analytics techniques serve as a powerful tool, unlocking a wealth of information and insights. These methodologies provide a depth of understanding that enables businesses to make well-informed, data-driven decisions.
1. Uncovering hidden trends with data mining
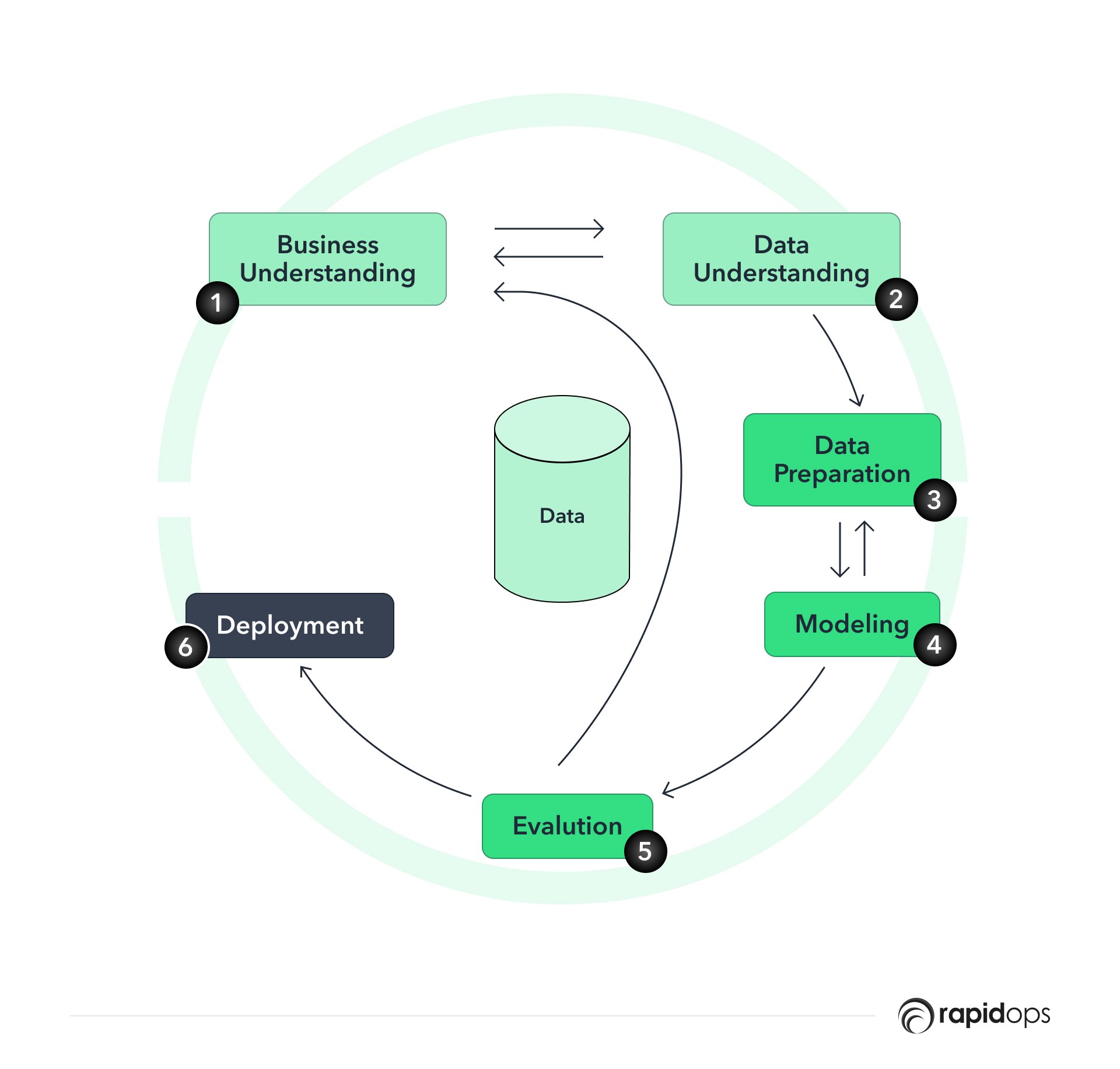
Data mining involves discovering large data sets' patterns, trends, and correlations. It can help businesses identify opportunities and risks that were previously unknown.
2. Predicting outcomes with regression analysis
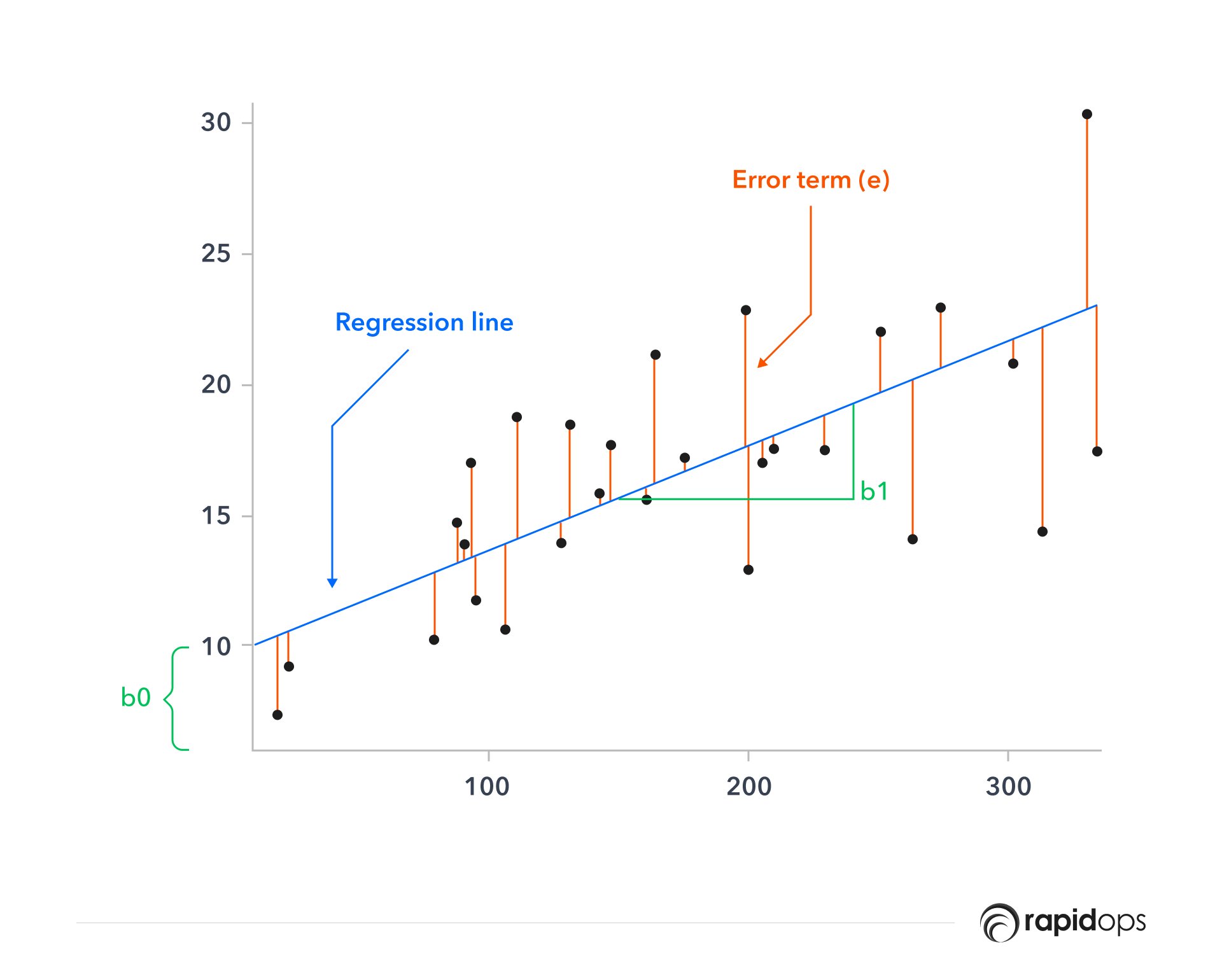
This statistical method helps businesses understand the relationship between dependent and independent variables. By modeling these relationships, organizations can predict outcomes and optimize processes.
3. Harnessing customer feedback through sentiment analysis
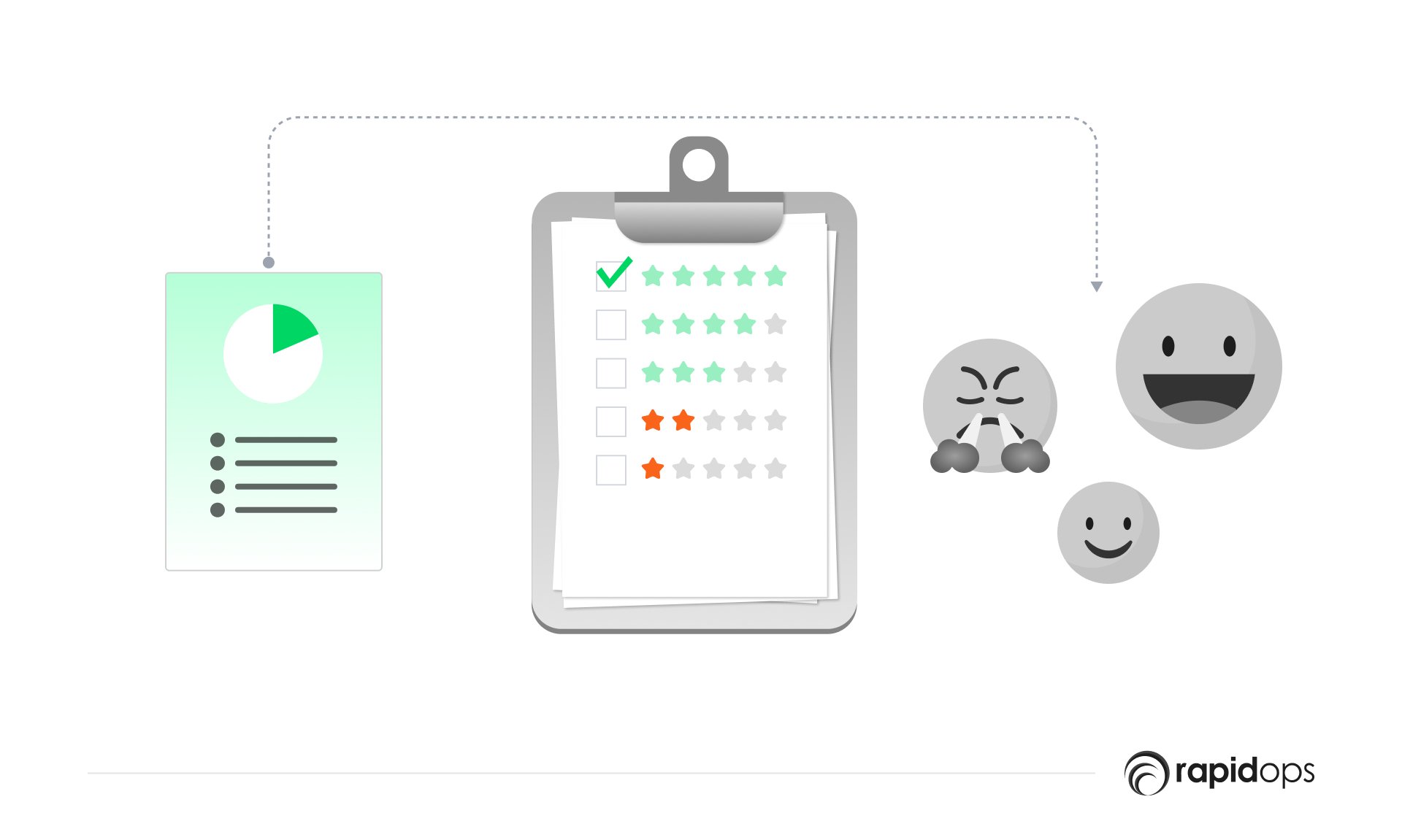
Sentiment analysis is extracting opinions, emotions, and attitudes from text data. It can help businesses understand customer opinions and preferences, enabling better decision-making and targeted marketing strategies.
4. Segmenting data using cluster analysis
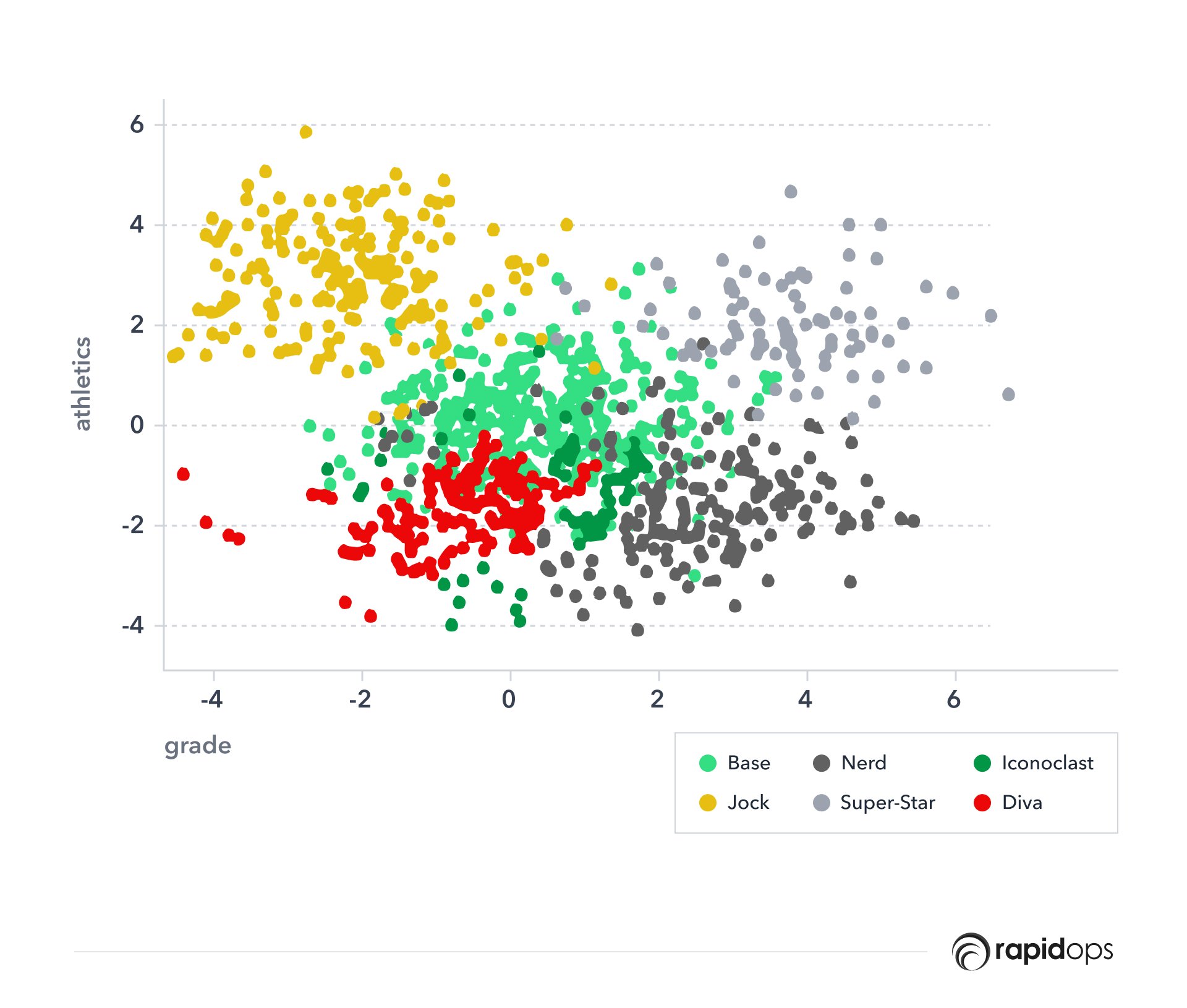
Cluster analysis is used to group similar data points based on their attributes. It can help businesses segment their customer base and develop tailored marketing strategies for each segment.
5. Automating decisions with machine learning
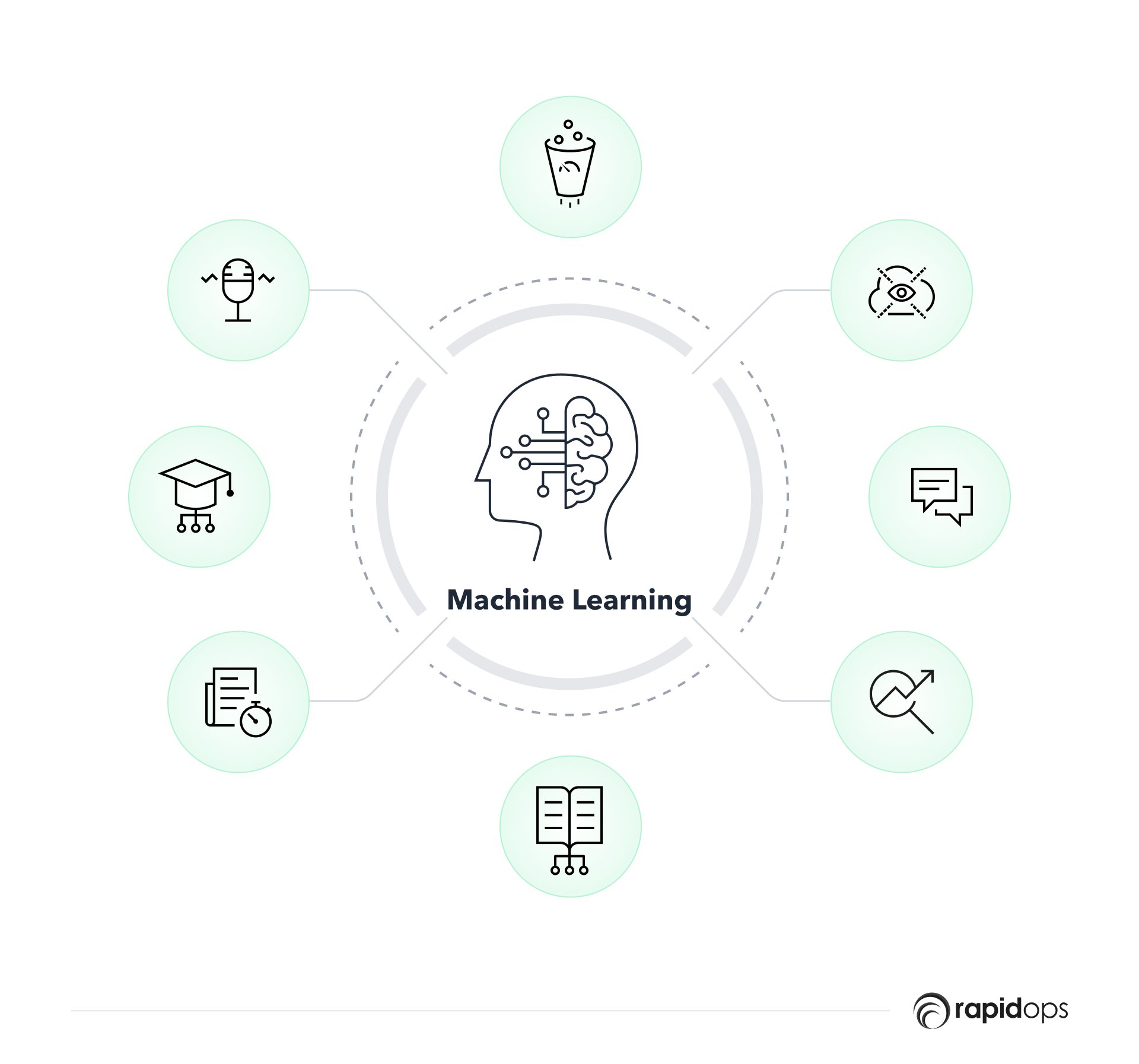
Machine learning is a subset of artificial intelligence that involves developing algorithms to learn from data. Businesses can use it to develop predictive models, automate decision-making, and optimize their processes.
6. Understanding consumer preferences with conjoint analysis
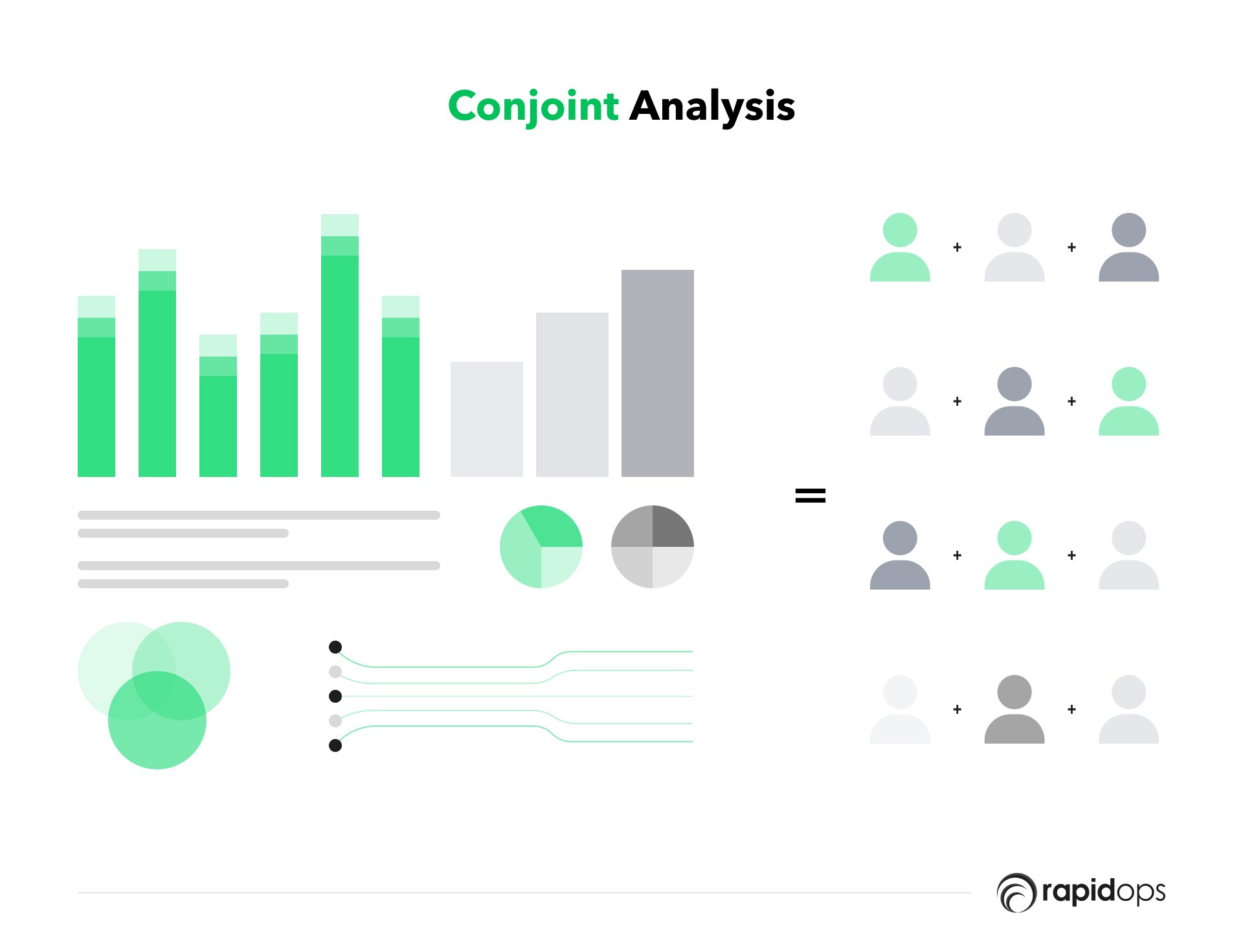
Conjoint analysis is a market research technique used to determine customer preferences and the relative importance of product features. It can help businesses develop products that meet customer needs and expectations.
7. Visualizing consumer perception through perceptual mapping
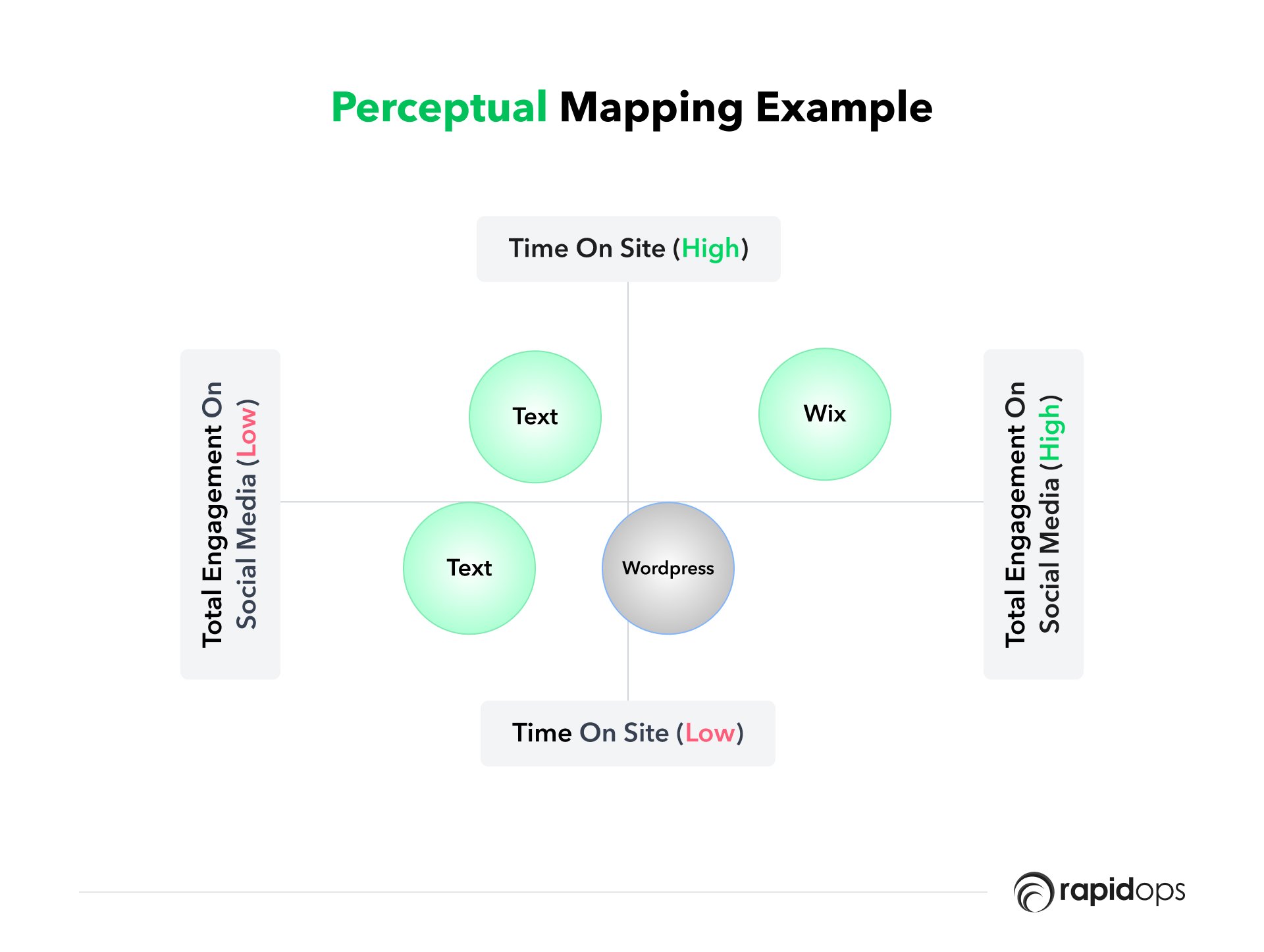
Perceptual mapping is a technique used to visualize how consumers perceive a brand or product relative to competitors. Businesses can use this information to develop competitive positioning strategies and improve product offerings.
8. Simplifying data complexity via factor analysis
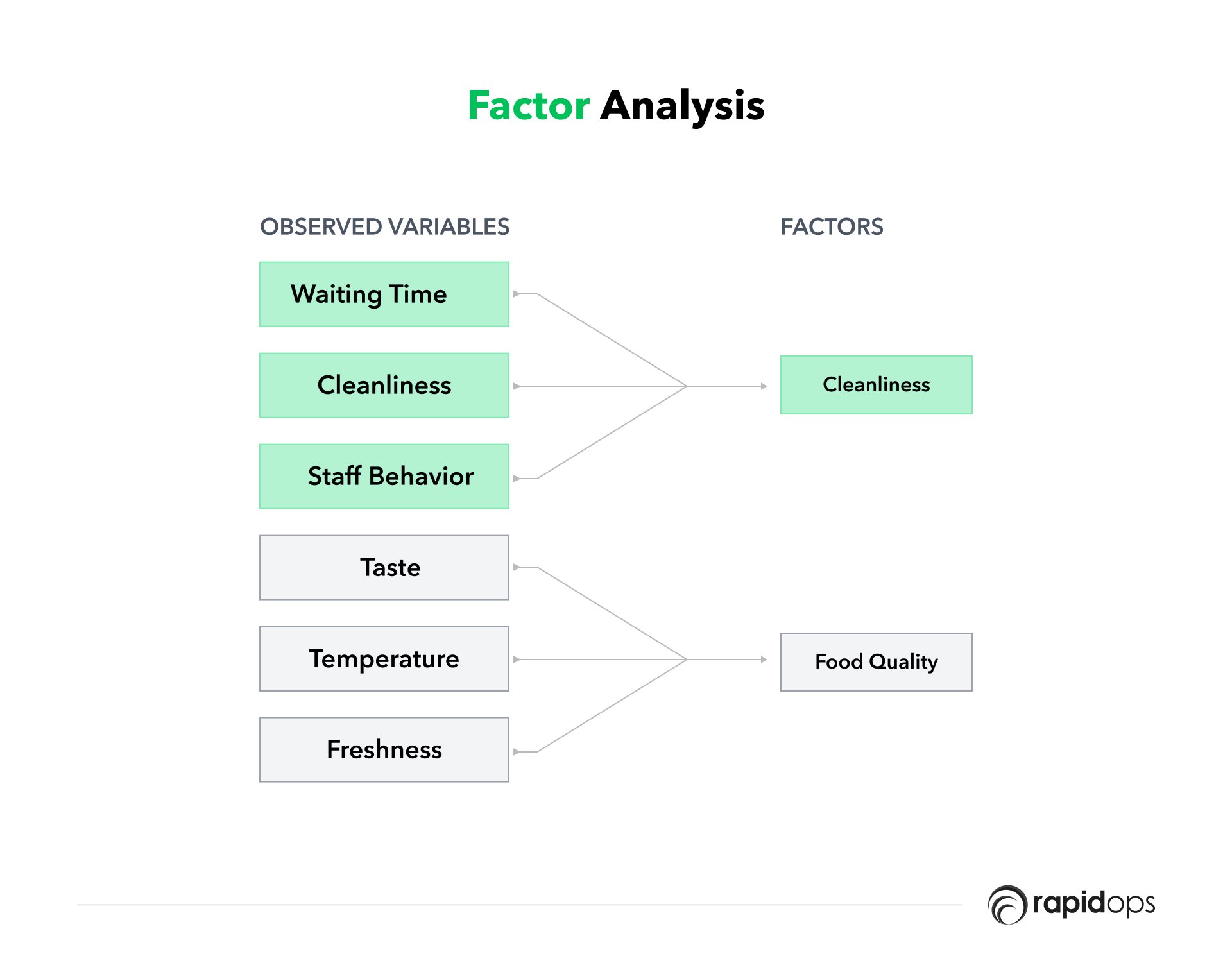
Factor analysis is a statistical method used to identify underlying relationships between variables. It can help businesses reduce data complexity and focus on the most critical factors driving success.
Building a successful data analytics strategy
A robust data analytics strategy is pivotal for driving innovation, enhancing decision-making, and bolstering overall business performance. Here, we guide you through the key steps to develop and implement a successful data analytics strategy.
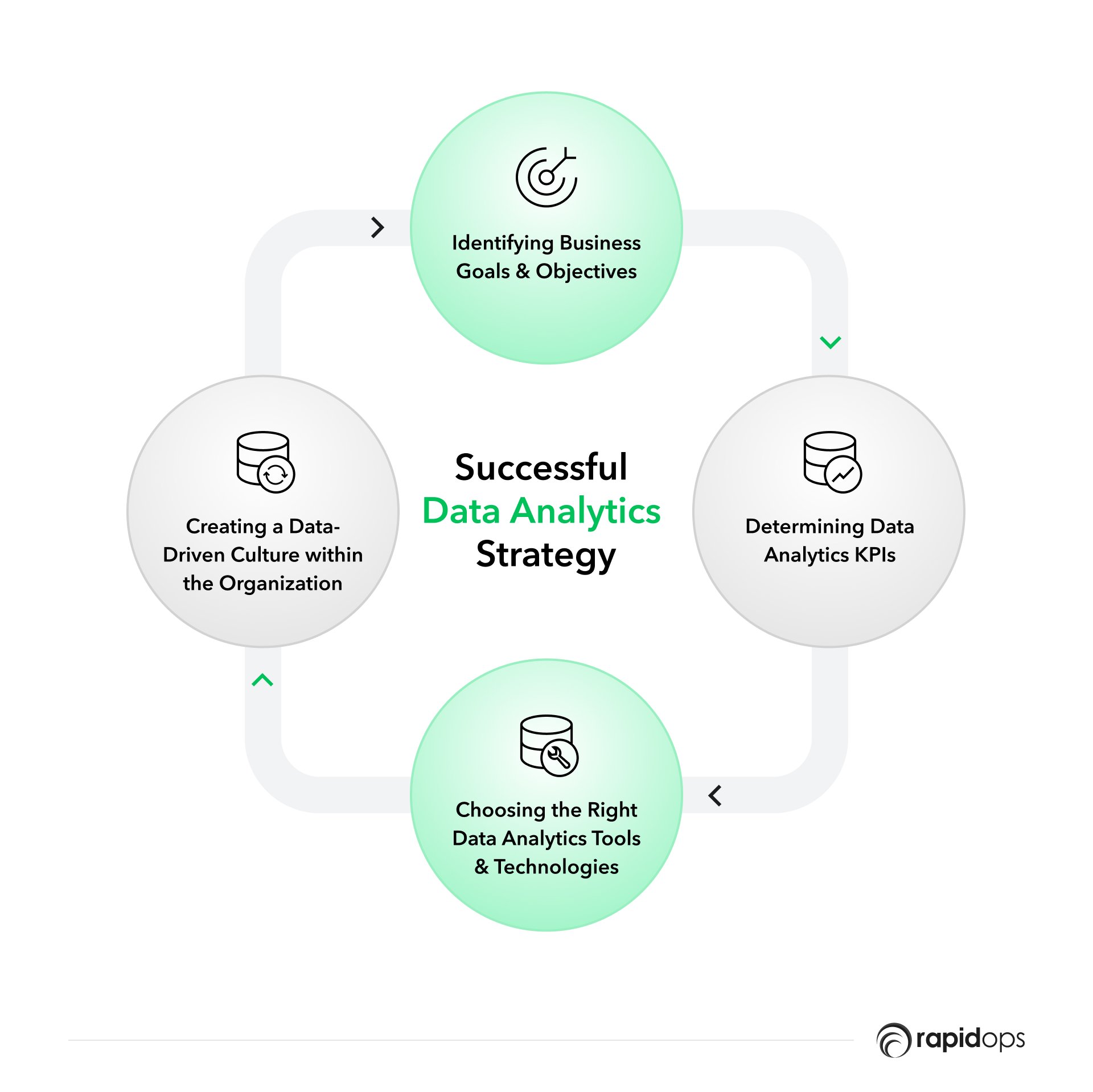
1. Identifying business goals and objectives
Start by defining the business problems you want to solve or the opportunities you want to explore using data analytics. This will help you align your data analytics efforts with your organization's broader goals.
2. Determining data analytics KPIs
Establish key performance indicators (KPIs) to measure the success of your data analytics initiatives. These KPIs should align with your business objectives and provide a clear benchmark for evaluating the effectiveness of your data-driven efforts.
3. Choosing the right data analytics tools and technologies
Select the appropriate tools and technologies that will support your data analytics strategy. Consider factors like ease of use, scalability, and integration with your existing systems.
4. Creating a data-driven culture within the organization
Fostering a data-driven culture within your organization is essential to maximize the benefits of data analytics. Encourage employees to leverage data in their decision-making processes and provide ongoing training and support to help them develop their data analytics skills.
Measuring the success of data analytics strategy
By prioritizing the evaluation, measurement, and continuous improvement of data analytics strategies, decision-makers can ensure that their organizations remain competitive and agile in the face of ever-changing market conditions. Let's dig deeper into the subject matter.
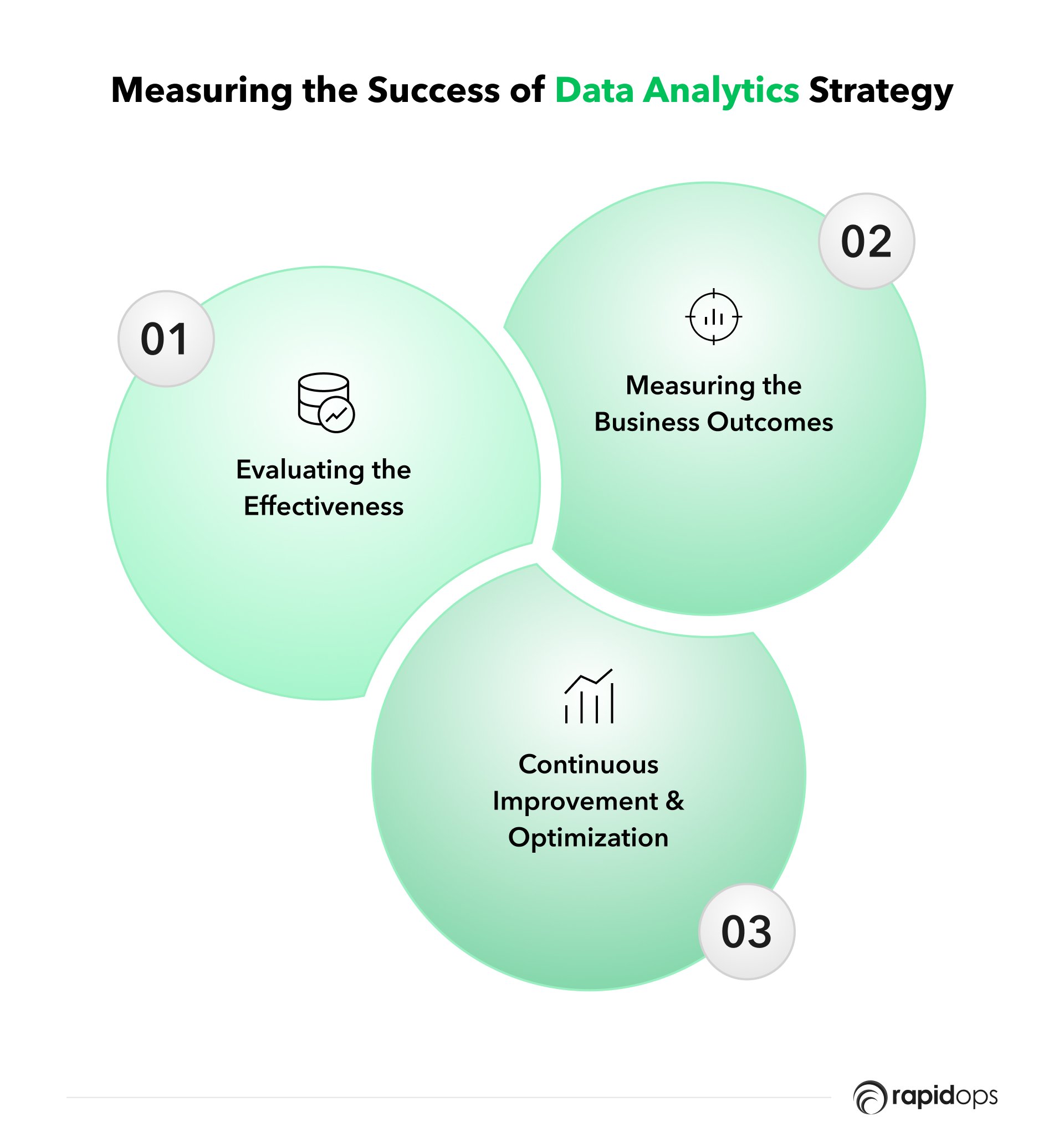
1. Evaluating the effectiveness of data analytics strategy
To evaluate the effectiveness of a data analytics strategy, organizations must first establish clear objectives and key performance indicators (KPIs) to measure progress. These KPIs should align with the organization's overall business goals and be quantifiable and actionable.
For example, a retail company aiming to improve customer retention might use metrics such as customer lifetime value, churn rate, and repeat purchase rate to evaluate the effectiveness of its data analytics strategy. By regularly monitoring these KPIs, decision-makers can determine whether the strategy delivers the desired results and adjust as needed.
Another aspect of evaluating effectiveness is comparing the organization's performance to industry benchmarks or competitor performance. This can help identify areas where the company is excelling or underperforming, informing adjustments to the data analytics strategy.
2. Measuring the impact of data analytics on business outcomes
Quantifying the impact of data analytics on business outcomes involves tracking the correlation between data-driven decisions and improvements in specific business metrics. By monitoring these metrics before and after implementing the data analytics strategy, organizations can determine the direct impact of their efforts.
For instance, a manufacturing company implementing data analytics to optimize production processes may see a reduction in production costs, a decrease in product defects, and an increase in overall production efficiency. By comparing these metrics before and after implementing the analytics strategy, the organization can quantify the impact of data analytics on their business outcomes.
Additionally, measuring the return on investment (ROI) of data analytics initiatives can help decision-makers assess the overall value of their strategy. This can be done by comparing the cost of implementing and maintaining the analytics strategy with the financial benefits it generates.
3. Continuous improvement and optimization of data analytics strategy
Continuous improvement is crucial to maintaining the effectiveness of a data analytics strategy. As market conditions, customer preferences, and business objectives evolve, so should the analytics strategy. Regularly reviewing the strategy's performance and adapting it to changing circumstances ensures that the organization continues to derive value from its data analytics initiatives.
For example, an e-commerce company using data analytics to optimize its pricing strategy should continuously test different pricing models and analyze the impact on sales, customer satisfaction, and profitability. By iterating on its strategy and incorporating new data and insights, the company can fine-tune its pricing approach to maximize revenue and customer satisfaction.
Taking the Next Step: Your advanced analytics journey with Rapidops
Rapidops Inc., with 14+ years of experience, is your trusted partner for advanced data analytics. Their experts help you choose the right analytics methods to optimize processes, make data-driven decisions, and gain a competitive edge. Contact us to start your advanced analytics journey today.
Frequently Asked Questions about advanced analytics
Several questions may arise in our journey to comprehend the complexities and benefits of advanced analytics in the business landscape. This FAQ section is designed to provide direct, easy-to-understand answers to some of the most common queries. Here, we’ll delve into the definition of advanced business analytics, its benefits to businesses, how it differs from traditional analytics, its role in various industries, and much more. Let’s address these inquiries one by one.
What are the business benefits of advanced analytics?
What are the 4 types of advanced analytics?
What is advanced analytics, in simple words?
What is the objective of advanced analytics?
What is the difference between analytics and advanced analytics?
What are the benefits of advanced analytics in the supply chain?
How do business analytics help business examples?
Why is business analytics becoming increasingly important in the business world?
What is the impact of business analytics on decision-making?
What’s Inside
- Importance of advanced analytics in business
- Benefits of advanced analytics in business
- Use cases of advanced analytics in business.
- Advanced data analytics techniques
- Building a successful data analytics strategy
- Measuring the success of data analytics strategy
- Taking the Next Step: Your advanced analytics journey with Rapidops